Data-Mining im B2B-Großhandel und Vertrieb
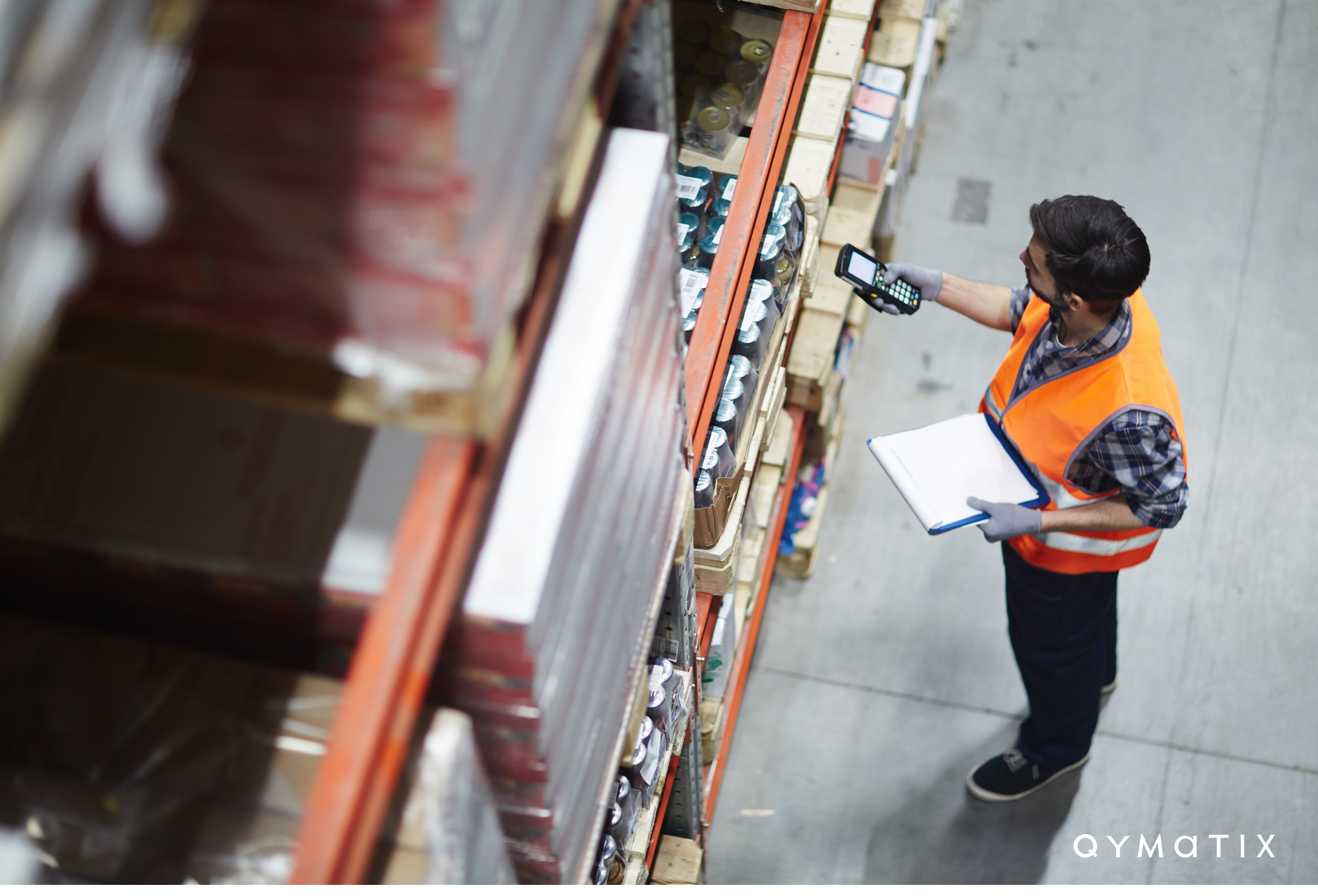
Wohin dürfen wir das PDF senden?
B2B-Vertriebsleiter und Geschäftsführer von Fachgroßhändlern in Deutschland stehen im heutigen wettbewerbsorientierten Geschäftsumfeld vor vielen Herausforderungen.
Der Aufstieg des E-Commerce macht es für Großhändler notwendig, Data Mining im ERP-System zu nutzen, um der Konkurrenz einen Schritt voraus zu sein.
Durch die Analyse der historischen Verkaufsdaten und die Implementierung von künstlicher Intelligenz in ihr ERP-System, können B2B-Großhändler wertvolle Einblicke in das Kundenverhalten gewinnen, die profitabelsten Produkte ermitteln und den optimalen Endpreis für jeden Kunden finden. Außerdem können sie Kunden an sich binden, bevor sie sie verlieren.
In diesem Artikel wird erörtert, wie wichtig Sales Data Mining für Großhändler ist und warum es ein Fehler wäre, zu lange damit zu warten. Legen wir los!
Die Bedeutung von Großhandelskundendaten
Reden wir nicht „um den heißen Brei herum“. Bei 1.000 bis 10.000 Kunden und 10.000 bis 100.000 Artikeln kann Ihnen nur künstliche Intelligenz sagen, welche Kunden mehr kaufen, weniger zahlen oder ganz abwandern werden. Aus diesem Grund nutzen erfolgreiche Großhändler ihre ERP-Systeme, um Verkaufsdaten zu analysieren und die Kundennachfrage vorherzusagen
Durch das Sammeln und Analysieren von historischen Verkaufstransaktionsdaten, können B2B-Vertriebsleiter Trends und Muster erkennen, die erst nach einiger Zeit sichtbar werden. Wenn Großhandelsunternehmen eine Predictive Sales Analytics Software mit Verkaufsdaten „füttern“, können sie z.B. die beliebtesten Produkte und Kategorien ermitteln und ihnen bei ihren Marketing- und Vertriebsbemühungen Vorrang einräumen. Darüber hinaus kann Data Mining im ERP-System dazu beitragen, die profitabelsten Kunden und Produkte zu ermitteln, was den Vertriebsleitern helfen kann, sich auf die Produkte und Kunden mit der höchsten Kaufwahrscheinlichkeit zu konzentrieren.
Außerdem kann Data Mining im Vertrieb dabei helfen, Muster im Kundenverhalten zu erkennen, z. B. akzeptable Preisspannen, durchschnittliche Kaufbeträge, Kaufhäufigkeit oder bevorzugte Produktbündel. Durch diese Erkenntnisse können Vertriebsleiter ihre Verkaufsstrategien auf die Bedürfnisse der einzelnen Kunden abstimmen, die Kundenzufriedenheit verbessern und den Umsatz steigern.
Die Vorteile von Data Mining für den B2B-Großhandel
Wie produktiv würden Sie sein, wenn Sie in die Zukunft schauen könnten? Das ist der Hauptvorteil einer Predictive Sales Analytics Software im Großhandel.
Data Mining im ERP für den B2B-Großhandel kann Vertriebsleitern und Geschäftsführern mehrere Vorteile bieten. Durch die Analyse der Großhandelskundendaten (ERP-Daten) können Händler die Kunden identifizieren, bei denen das Risiko der Abwanderung oder des Wechsels zu einem Mitbewerber am höchsten ist. Mit diesen Informationen können Vertriebsleiter Kunden proaktiv an sich binden, indem sie Anreize für Wiederholungskäufe schaffen, personalisierte Rabatte anbieten und gezielte Marketingkampagnen erstellen.
Durch das Data Mining im ERP-System können Vertriebsleiter auch Cross-Selling- und Upselling-Möglichkeiten erkennen. Denn Data Mining im ERP analysiert die Kaufhistorie ihrer Kunden und im B2B-E-Commerce. Bei Tausenden von Kunden und Produkten bieten diese Informationen einen erheblichen Wert. Eine KI-basierte Predictive Sales Software kann diesen Prozess automatisieren.
Darüber hinaus kann die Analyse von Kundendaten dabei helfen, Kunden zu identifizieren, die in Zukunft wertvoll werden. Indem sie ihnen Priorität einräumen, können sich die Vertriebsleiter darauf konzentrieren, diese Kunden zu halten, indem sie ihnen den bestmöglichen Service und Anreize bieten. Auf diese Weise können sie einen loyalen Kundenstamm aufbauen, der den Umsatz steigert und als Botschafter der Marke fungiert, indem er sie unter Gleichgesinnten bewirbt.
Der Bedarf an einer standardisierten Predictive Analytics Software für den Vertrieb
Versuchen Sie nicht, das Rad neu zu erfinden. Um die Möglichkeiten des Data Mining im ERP voll auszuschöpfen, sollten B2B-Vertriebsleiter eine Predictive Sales Software für den Vertrieb einsetzen. Predictive Analytics (DE: Prädiktive Analysen) können Händlern dabei helfen, das Kundenverhalten vorherzusagen und die Produkte zu identifizieren, die sich am ehesten verkaufen lassen.
Darüber hinaus kann eine Predictive Sales Software Vertriebsmanagern helfen, den optimalen Preispunkt für jeden Kunden zu bestimmen, was dazu beitragen kann, den Gewinn zu maximieren und gleichzeitig Kunden zu binden. Mithilfe von einer prädiktiven Standardsoftware können Vertriebsleiter wertvolle Erkenntnisse über das Kundenverhalten gewinnen und datengestützte Entscheidungen treffen, um Umsatz und Gewinn zu steigern.
Auch bei der Planung der Nachfrage und der Optimierung des Lagerbestands kann eine Predictive Analytics Software den Vertriebsleitern unter die Arme greifen. Durch Vorhersagen, welche Produkte sich wann verkaufen werden, können sie Lagerausfälle oder Überbestände vermeiden, die für das Unternehmen kostspielig sein können. Die richtigen Produkte auf Lager zu haben und sie zu einem angemessenen Preis anzubieten, kann außerdem die Kundenzufriedenheit verbessern, die Lieferzeiten verkürzen und den Ruf des Unternehmens verbessern.
Best Practices für das Sales Data Mining im ERP-System
Sales Data Mining kann ein komplexer Prozess sein, der einen systematischen Ansatz erfordert, um effektiv zu sein. Um die Daten von Großhandelskunden optimal zu nutzen, sollten B2B-Vertriebsleiter diese Best Practices befolgen:
Sammeln Sie saubere Daten: Stellen Sie sicher, dass Ihre Daten korrekt, vollständig und aktuell sind. Nutzen Sie automatisierte Prozesse zur Erfassung und Bereinigung der Daten, um Fehler und Unstimmigkeiten zu minimieren. Beginnen Sie mit den zuverlässigsten Daten, Ihren ERP-Daten.
Führen Sie die Daten einer Predictive Sales Software zu. Diese Großhandelssoftware setzt statistische Methoden, maschinelles Lernen und Datenvisualisierungstools ein, um die Daten zu analysieren und Muster, Trends und Korrelationen zu erkennen.
Erstellen Sie eine datengesteuerte Strategie: Nutzen Sie die Erkenntnisse aus der Datenanalyse, um eine datenbasierte, auf die Bedürfnisse Ihrer Kunden zugeschnittene Verkaufsstrategie zu entwickeln.
Geben Sie die neu entdeckten Chancen an Ihre Vertriebsmitarbeiter weiter und stellen Sie sicher, dass deren Kundensegmente und Fälle korrekt angewendet werden.
BERECHNEN SIE JETZT DEN ROI VON QYMATIX PREDICTIVE SALES SOFTWARE
Data Mining ERP im B2B-Großhandel & Vertrieb – Fazit
Im heutigen wettbewerbsorientierten Geschäftsumfeld sollten Großhandelsunternehmen, mit 1.000 bis 10.000 Kunden und 10.000 bis 100.000 Artikeln, das ERP-Data Mining als eine wichtige Investition erkennen.
Die gute Nachricht: Es gibt eine Standardsoftware, die ihnen dabei helfen kann: Eine Predictive Sales Software überwacht und analysiert automatisch die Daten von B2B-Großhandelskunden. So können Vertriebsleiter wertvolle Erkenntnisse über das Kundenverhalten gewinnen, die profitabelsten Produkte identifizieren und den optimalen Endpreis für jeden Kunden finden. Außerdem können sie Kunden an sich binden, bevor sie sie verlieren.
Um die Möglichkeiten des Data Mining im ERP-System voll auszuschöpfen, ist eine Predictive Sales Software der Schlüssel dazu. Best Practices für das Sales Data Mining in ERP-Systemen ermöglichen Vertriebsleitern eine datenbasierte, auf die Bedürfnisse ihrer Kunden zugeschnittene Vertriebsstrategie. So können sie die Kundenzufriedenheit verbessern und gleichzeitig Umsatz und Gewinn steigern.