Why Projects using AI in B2B Sales fail and yours will too
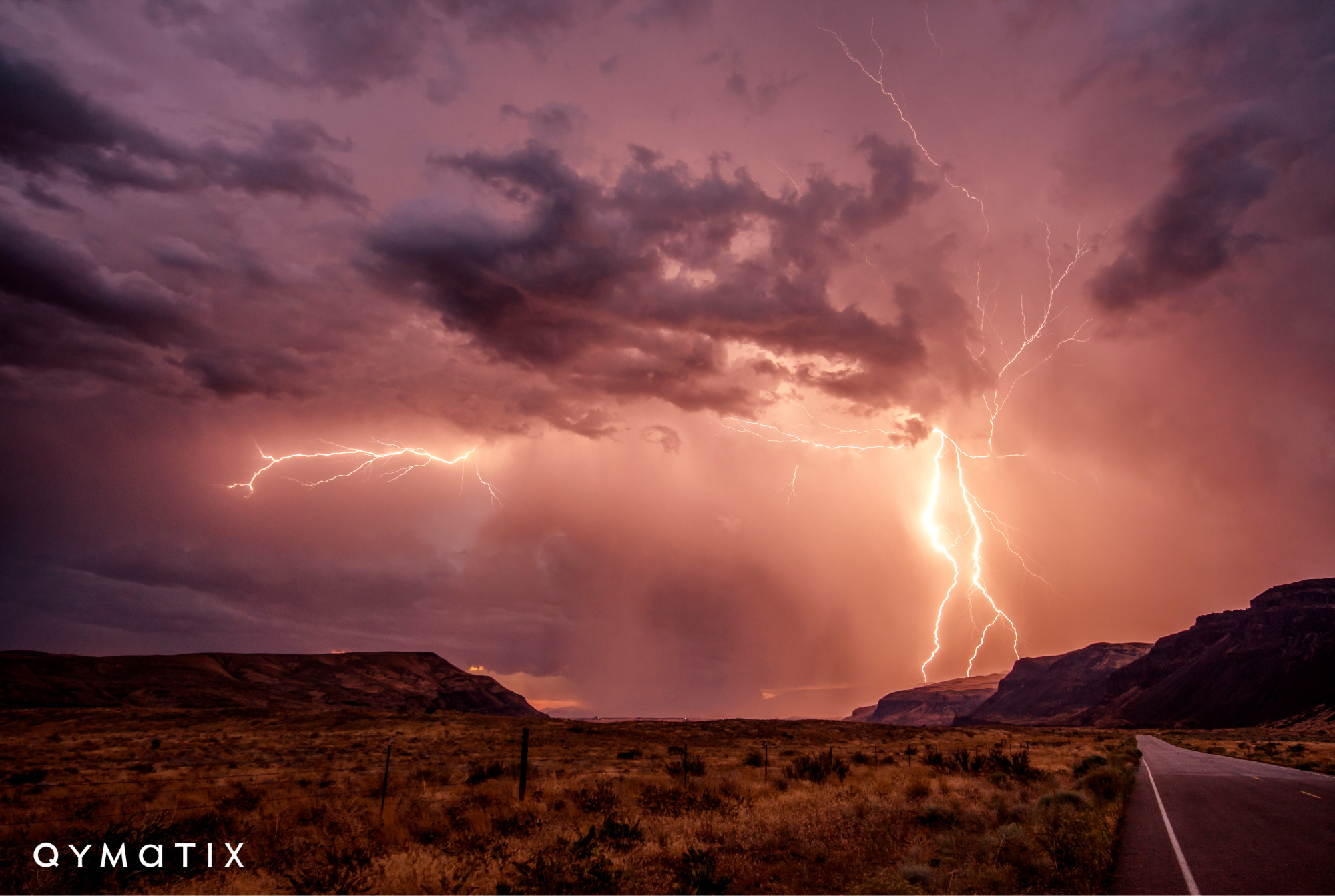
Successful organizations do not underestimate the complexity and hidden costs of artificial intelligence (AI) projects.
Artificial intelligence (AI) for sales has become an arms race. Correctly employing it will separate the winners from the losers in Business-to-Business (B2B). There are, however, very high chances that your AI project will not deliver the promised ROI or will ultimately fail.
Nick Heudecker, a long time Gartner Vice-President in Data & Analytics, estimated that over 85% of projects in data science fail. Several studies support his findings in AI for Sales. For example, Diane Hagglund, founder of Dimensional Research, found that less than 1 in 20 companies successfully deployed machine learning to a production environment. In 2017, the CIO magazine stated a 30% failure rate for AI in B2B.
Based on our own experience deploying our Predictive Sales Software using AI and often competing against internal AI projects, there are three main reasons why they fail:
Understated sales complexity, Data Scientist’s wishful thinking,Poor ERP data quality for AI and missing informative CRM data.
These three reasons lead to failure to deliver business value and erode trust in AI technologies. Notwithstanding developments in AI technology and infrastructure, the sad record-track of AI projects does not seem to have improved much in the twenty years.
Allow me to review them in detail.
Reason Number One: Understated sales complexity
Artificial intelligence in B2B sales does not occur in a vacuum. It is part of orchestration and makes sales processes more efficient. Sadly, many data scientist or business intelligence managers in charge of the internal AI development play down the caveats of their sales operations.
The project success depends on the adoption and acceptance by the management, salespeople, decision-makers and even customers. Unfortunately, companies sometimes fail to grasp what an AI solution would require and look once implemented. That is one of the main reasons why often internal AI projects fail. Therefore, keeping the focus of the solution is critical.
On the other hand, if your project is too broadly defined, with many stakeholders having a saying on the table, the odds are against it. Too many interfering voices in any project make it a risky enterprise.
How do successful companies solve this dilemma? By carefully deciding when to make and when to buy.
Make, if AI will be a core part of your business, such as making your products more intelligent. Then, buy, for supporting functions, such as sales or marketing.
Besides, sadly but often, companies implement an AI solution to make their salespeople faster “order-takers” instead of offering them an aiding tool. In other words, they do not provide their sales teams with a solution to make them more successful, only an excuse to make them repeat ineffective tasks faster.
B2B sales are complex. Your AI solution should not. Successful sales leaders chose an AI-based Predictive Sales Software that reflects their Sales Ops complexity instead of risking an expensive and ineffective AI project.
Sales is not easy, yet your AI sales solution does not need to be complex.
Reason Number Two: Data Scientist’s wishful thinking
Finding data scientists is extremely hard and expensive nowadays. Studies show that for each one hired, 43 positions are still vacant. Therefore, as a company, you should invest their time in the most efficient possible way.
Data scientists usually spend weeks gathering and cleaning data, selecting features and models, designing production environments, and developing the infrastructure needed to run an AI solution continuously. However, there are tools in the market that can perform these tasks in a few minutes.
Sadly, many companies charge developing AI for sales to their internal data science team or business intelligence department. Both have an incentive to keep the ball running for as long as possible, even when the AI project has meagre chances of success. Many refuse to buy an AI solution for sales, simply out of a habit of avoiding “not invented here.”
I call this “a data scientist’s wishful thinking”. If they had enough data, more time, or if the sales team would use their models, the AI project would succeed. Instead, in both cases, they manage to deflect the blame on someone else while keeping resources invested.
Data quality always offers room for improvement and is a field where your data owners can work extensively. On the other hand, only collaborative machine learning models can help if there is not enough data. Lastly, your sales team will employ AI if they have the incentives to do it and the AI software supports them.
Companies that successfully apply artificial intelligence in sales invest their resources wisely.
Reason Number Three: Poor ERP data quality for AI and missing informative CRM data
You cannot use artificial intelligence in sales if you do not have enough sales data. If your business has a limited data set, few customers, and transactions, carefully assess your expectations with an expert partner.
In general, how much data is enough for AI? It depends on the case and the technology. For example, in the case of AI for sales, 1.000 customers with around 10.000 transactions per year are maybe the lowest number of records you can properly use. Furthermore, for such a small data set, only collaborative machine learning models might enable continuous intelligence.
Similarly, a minimum degree of CRM and ERP data quality is the foundation for Artificial Intelligence in Sales. If your sales data cannot satisfy a modicum ERP data quality or valuable CRM features are missing, the return on investment of AI in B2B sales [LINK TO SOMETHING] will suffer.
Garbage in, garbage out. Many AI projects in Sales fail because companies do not know how to define data quality or where to invest. Improving data quality is always a matter of cost-benefits. Having a duplicated customer or product erases most of the information AI can obtain about them.
Not all CRM data offers the same information gain. In my experience with a couple of billion sales transactions, there are usually a handful of valuable data sets, some characteristics where it makes sense to invest and some where the ROI is unattractive. For example, investing in ERP data quality actions such as product typology and avoiding duplicated accounts improve the data available to an AI-Solution in sales significantly.
On the other hand, using outdated data, duplicates, incorrect or missing information will frustrate your team and probably lead to failure.
So my bottom line is the following: no company has perfect data, yet the better the data, the greater the chances of success.
CALCULATE NOW THE ROI OF QYMATIX PREDICTIVE SALES SOFTWARE
Why AI Projects in B2B Sales fail and yours will too – Summary.
Artificial intelligence is transforming B2B sales, and companies will keep investing in it. Everyone in the company wishes the AI project to succeed; some will, many will not. Successful managers focus on projects with a higher chance of delivering a positive ROI and employ standard AI software when possible.
Sadly, when companies develop AI software for B2B sales internally, the data science team has all incentives to understate the complexity of the sale operations. As a result, they mainly sweat to find any machine learning model that works without realizing or stating that the model is just one piece overall.
The team tasked with developing an AI solution for sales will put effort and work hard for long months to try to overcome the unstated complexities, wishing them away. But, most likely than not, the project will not survive the following change of priorities and will be stopped without have realized any value.
One cannot entirely blame the technical team for the failure of the AI project not. Poor ERP data quality and missing informative CRM data both play a part. Companies are better off by investing in those areas and using standard AI software for sales.
Do not let a bad experience with AI for Sales make you miss out on the great value it can create in your company.
I WANT PREDICTIVE ANALYTICS FOR B2B SALES.
Further Read:
Gartner (2020): Five Questions for a Successful AI Project
Harvard Business Review (2019): Article Technology Building the AI-Powered Organization
Nechu BM (2019): Why 85% of AI projects fail
Neoteric (2020): The single most important reason why AI projects fail
Ryohei Fujimaki (2020): Most Data Science Projects Fail, But Yours Doesn’t Have To