Four facts about predictive analytics that you should know today
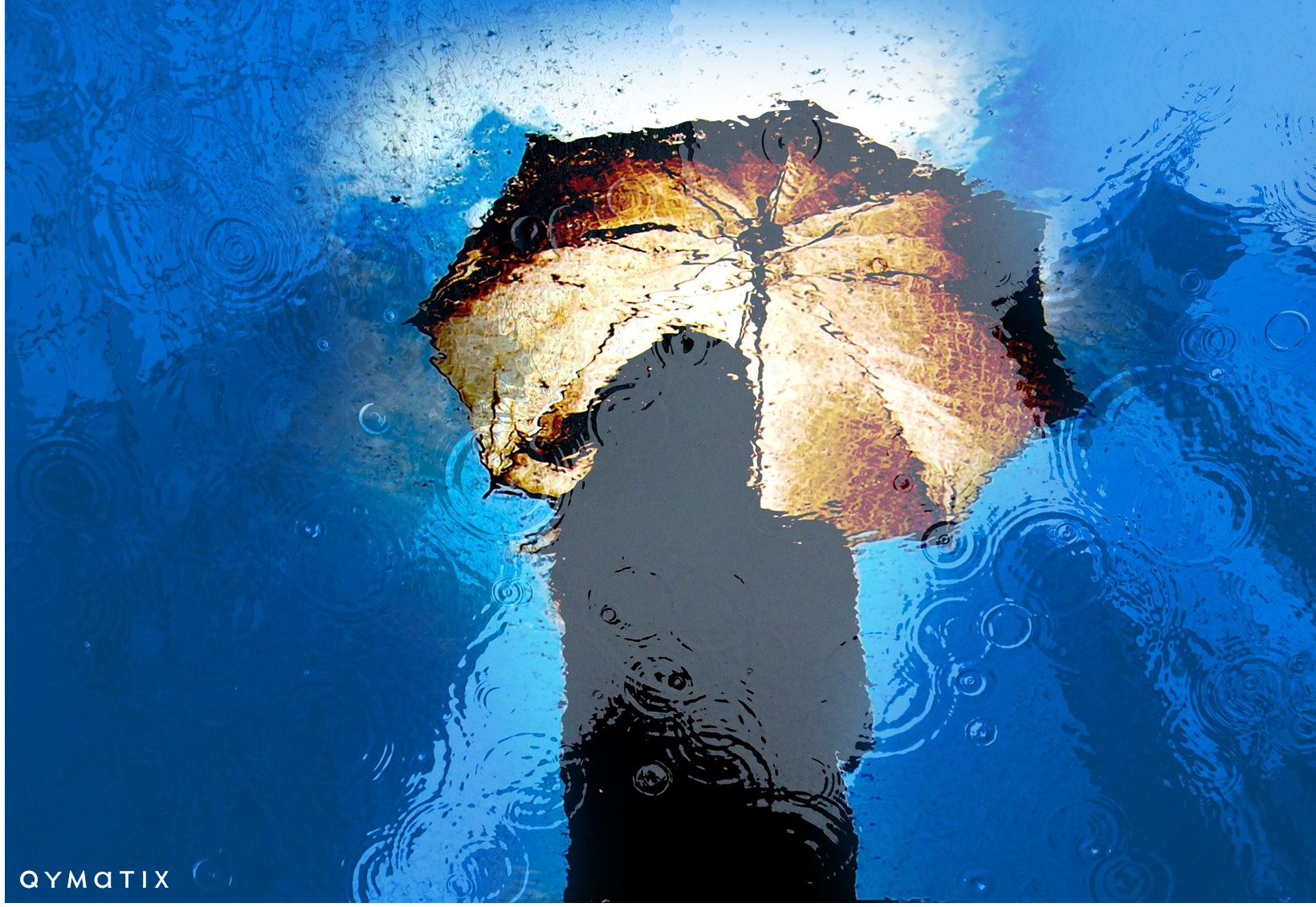
Please enter your Email address
Predictive Analytics and AI – If you want to use this technology, you should first understand it.
If you keep up to date with new technologies, you have undoubtedly come across several professional articles about Predictive Analytics (PA).
According to the Harvard Business Review, experts agree that predictive analytics delivers a significant gain from your data: actionable information. Precise forecasts are the holy grail for sales decisions in the B2B sector.
But anyone who wants to use predictive analytics profitably should also understand it. For this reason, we have compiled four facts about PA that are often underestimated or ignored in practice.
As a quick refresher: Predictive analytics is a technology that learns from historical data to predict future events.
It is a technology to look into the future.
A relevant example for B2B sales would be the prediction of customer churn – before the customer has left.
1. Predictive analytics is not always right.
Nobody knows the future – not even super-smart analyses. That is a fact. So why all the effort? The keyword is “probabilities”.
Predictive analytics calculates the probabilities of various future events. And it does quite accurately, too. With new technologies, you can achieve a 90% hit rate. So, the technology tries to get as close as possible to the real future. If someone or something tells you that an event will happen “100 % probability” they are not using predictive analytics.
A forecast, per definition, lays in the future and cannot be always right.
There are two types of forecasting errors. “False positive” and “false negative”. Let´s see what it means by a simplified weather forecast:
The picture shows on the vertical axis, the forecast, and on the horizontal axis, the actual weather.
That results in two forecast errors: The model says “it should be raining”, but it is not raining. That would be “false positive”. The opposite is, obviously a “false negative” (the model says it should not be raining, but it is).
A PA-Model can usually minimize one error at the expense of the other error. That means, if you want less “false positive” results, there will automatically be more “false negative” forecasts.
The question is: which error is worse? In our weather example, the consequences of a “false positive” would be that you carry an umbrella with you all day and don’t need it.
The consequences of a “false negative”, however, are that you might get wet, get ill and miss work. How much more than carrying an umbrella would that cost you?
For this reason, you can expect that weather forecasts are always a bit more “pessimistic” to avoid a “false negative” (and people getting sick).
2. Predictive analytics is not just one model.
Talking about umbrellas. Predictive analytics is an umbrella term for a set of models, to predict the future from the past. It starts with elementary principles.
Let’s stay with the weather:
The following assumptions are plausible (and often not even too wrong) ways to predict the weather:
“The weather tomorrow will be like tomorrow one year ago”.
Or, “tomorrow it will be like today.”.
Of what else can you think?
“Tomorrow it will be as much colder by as many degrees as from yesterday to today.”
These simple statements are already (very basic) models and fall under the term “Predictive Analytics”.
Fortunately, there are somewhat more complex (and hopefully more accurate) models and algorithms from statistics that produce exact forecasts based on thousands of characteristics and data.
3. Artificial Intelligence (AI) and Predictive Analytics strengthen each other.
Are you confused? Now, what is the difference between predictive analytics and artificial intelligence (AI); Is predictive analytics always AI?
No, predictive analytics and artificial intelligence are two different disciplines. However, there is an overlap. In this overlap, methods of artificial intelligence (more precisely, machine learning) optimize forecasts.
According to a study by the Business of Fashion platform, AI-based algorithms reduce forecasting errors by up to 50%. It means that AI and machine learning have a much higher hit rate than static, rule-based forecasting models.
How can a Predictive Analytics Software work for me?
4. Predictive Analytics has many applications.
In principle, you can use Predictive Analytics (PA) wherever historical data is available. However, a further prerequisite is that there must be specific patterns in the data.
We were once asked in a project whether our software can predict the lottery numbers. I wish – then we would probably no longer be a Startup.
No, we cannot predict the lottery. Lottery numbers are absolute random. If there are correlations in the history of the lottery numbers, they are only fake, spurious correlations. To get the lottery numbers right, you would need a glass ball and supernatural abilities. Or a lot of luck.
Thus, gambling does not fall under the scope of PA.
No more than saying, that there is almost a 50% chance of getting a red in the roulette (think about the zero).
One of the best-known areas of application and a pioneer of predictive analytics is probably the insurance industry. Based on personal characteristics, you can calculate probabilities for later occurring illnesses or even accidents. How useful is that, if you have to decide whether to insurance someone?
But that is, of course not all. For B2B sales alone, there are many cases for predictive analytics.
We have often found that our customers initially only understand predictive analytics as cross-selling (i.e., suggesting additional products that a customer might buy).
That is understandable because Amazon’s suggestions “other customers also bought…” or “This could be interesting for you…” make the cross-selling function very popular.
But a recommender system is not the only thing you can use predictive analytics for:
You can make price suggestions with the highest probability of acceptance per customer and product. Also known as “Dynamic Pricing”.
Or the PA-Model can draw your attention to customers who are most likely to switch to your competitor. The technical term for this is “churn prediction”.
The list is even longer. Once you have historical data on something, PA-Models can make predictions. Leads with the highest likelihood of purchase, inventory forecasts, or offers your customers will most likely accept.
People also use Predictive analytics outside of B2B sales, such as by the police (predictive policing).
CALCULATE NOW THE ROI OF QYMATIX PREDICTIVE SALES SOFTWARE
Four facts about predictive analytics – Summary
Why is it so important to know a few basic facts about Predictive Analytics (PA)?
Predictive Analytics has a wide range of applications. So, it may well be that you too are confronted with this topic in your working environment.
Primarily if you work in B2B sales, you can be sure that predictive analytics will come to you.
And when it does, the success of your Predictive Sales Software will also depend on you and how you deal with it. Now you know that forecasts cannot always be right – but you also know that over hundreds of predictions, a clear majority hit the mark.
What you also know is that there are AI-based PA-Models and non-AI-based models (these are manual and rule-based). Artificial intelligence has dramatically optimized conventional PA models.
Now you´re ready for your own experiences with Predictive Analytics! Good Luck.
I WANT A PREDICTIVE SALES ANALYTICS SOFTWARE!
Further Read:
McKinsey & Business of Fashion (2018): AI Gets Real.
Theo Kieschnick (2019): Predictive Analytics. Ed.: Trend Report. (German language)