The Importance of Recommendation Systems Nowadays
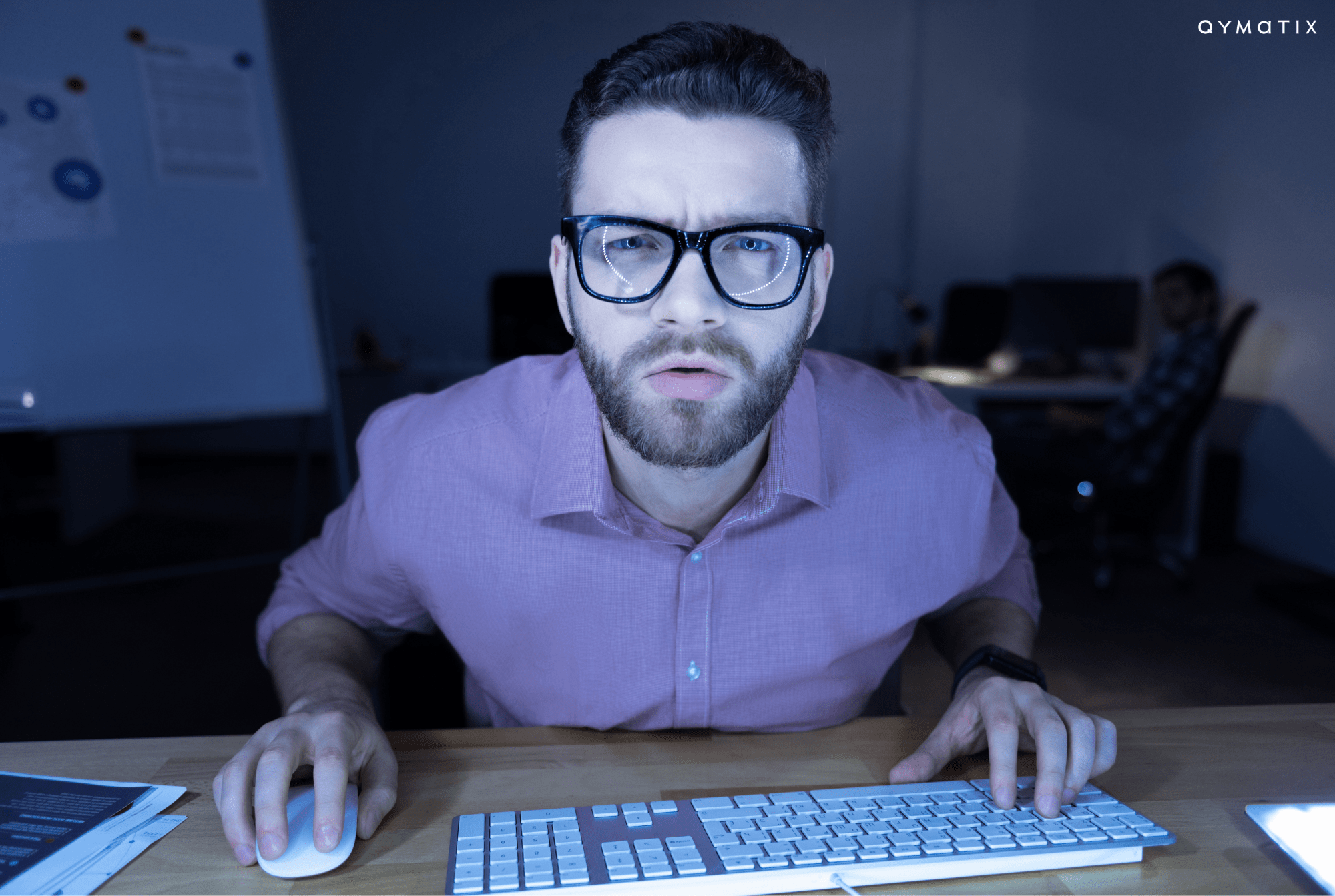
How do recommendation systems work and why are they important for B2B companies?
In recent years, the rapid use of the internet has made it clear that it is the most popular for communication and product purchases. As a result, the amount of available digital information has been increased.
This situation has created the challenge of information overload which hinders timely access to items of interest on the Internet. Although the so-called information retrieval systems, such as Google, are a partial solution, these systems are unable to map available content to user’s interests and preferences.
The result of this absence of prioritization and personalization led to the increase of demand for recommendation systems. In this article we explain what recommendation (or recommender) systems are, how they work and how helpful can be for companies, even for small ones.
What is a Recommendation System?
Although several definitions about recommender systems have been given, we could define them as information filtering systems that tackle the problem of information overload. They filter information according to the user’s interest and preferences or observed behavior about item.
So, based on the user’s profile, these systems can predict whether a product will be preferable by a user or not. More broadly, recommender systems represent user preferences for the purpose of suggesting items to purchase or examine and are now an integral part of a lot of e-commerce sites.
How do Recommender Systems Work?
There are many different types of techniques and implementations out there. The most famous recommender systems work in two ways.
1) Content-Based Filtering
In the first case, content-based filtering is a domain-dependent algorithm. Its essence is to filter or sort recommended items by matching or relevance between item and user features. It mainly depends on the user and in particularly his related information and the related operating behavior.
A statement that we could use to describe these systems could be “Show me more of the same of what I’ve liked before.”
These techniques make recommendations by learning the underlying model with either statistical analysis or machine learning techniques. One advantage of this technique is that the profile of other users is not needed since the preferences of other users will not affect others. Moreover, if we have a new user or the user profile changes, this technique still has the potential to adjust its recommendations within a very short period of time. As a disadvantage we could mention the so-called limited content analysis. That means, rich description of items and very well-organized user profile are needed so as recommendations can be made to users.
2) Collaborative Filtering
The second approach is the most mature and the most implemented. It is the collaborative filtering where the interactions between users and items from the past are stored in the so-called “user-item interactions matrix”. Then, users with relevant interests build a group called “neighborhood” after calculating similarities between their profiles. After that, a user gets recommendations to those items that he has not rated before but that were already positively rated by users in his neighborhood. A statement for this technique would be ‘Tell me what’s popular among my neighbours because I might like it too’.
In general, collaborative filtering can be divided into two categories: memory-based and model-based.
The first one has two characteristics:
– It uses statistical techniques to approximate user or items , like the cosine distance or the pearson correlation.
– It uses the entire user-item dataset to generate a recommendation.
On the other hand, the model-based recommender system:
– It develops a model of users to learn their preferences, and
– the models can be created using Machine Learning techniques like f.e. regression, clustering and classification.
One major advantage of collaborative filtering is that it can perform well even if there is not much content associated with items or it is difficult for a computer system to analyze it (such as opinions). In addition, this technique can recommend items that are relevant to the user although the content is not in the user’s profile.
Why Recommendation Systems Are Important.
1. They DO Work.
Amazon, one the the most famous companies around the world has spent over 10 years in developing a recommender system for their needs. As a result, the additional sales were about another 20% from recommendations in 2002.
Moreover, Netflix established a competition in 2009 to improve the accuracy of its movie recommender system by 10%, indicating how much important a recommender system can be for the success of a company. Already, 35 percent of what consumers purchase on Amazon and more than a half of what they watch on Netflix come from recommendations systems.
2) Recommender systems can significantly enhance user likelihood to buy the items recommended to them, the loyalty and their overall satisfaction.
3) They reduce transaction costs of finding and selecting items in an online shopping environment.
4) Since they serve to reduce consumer search costs and uncertainty associated with the purchase of unfamiliar products, recommendation systems offer an improvement regarding decision making process and quality.
5) Recommender systems can significantly improve a company’s revenue as they play a key role in cross selling. They make it possible for companies to ensure that the customer regularly discovers new products that may be of interest to him. The ideal situation is one where your existing customer is not aware of a product or service that would improve their customer experience. From this point of view, it is very likely that clients will return to the provider and recommend it to others.
CALCULATE NOW THE ROI OF QYMATIX PREDICTIVE SALES SOFTWARE
The Importance of Recommendation Systems Nowadays – Conclusion
In general, recommendation systems can recognize patterns regarding similar products and users that even the best sellers of the companies cannot do. This ability of the recommendation systems is indisputable.
On the other hand, the experience of a company’s salespeople in approaching customers and their ability to understand the requirements of the time is not only useful but valuable as well. Thus, it becomes clear that their combination is essential for a successful product sales policy, customer satisfaction as well as attracting new ones.
I WANT PREDICTIVE ANALYTICS FOR B2B SALES.
Further Read:
Ian MacKenzie et. al. (2013): How retailers can keep up with consumers. Ed.: McKinsey