The Evolution of Artificial Intelligence and Machine Learning
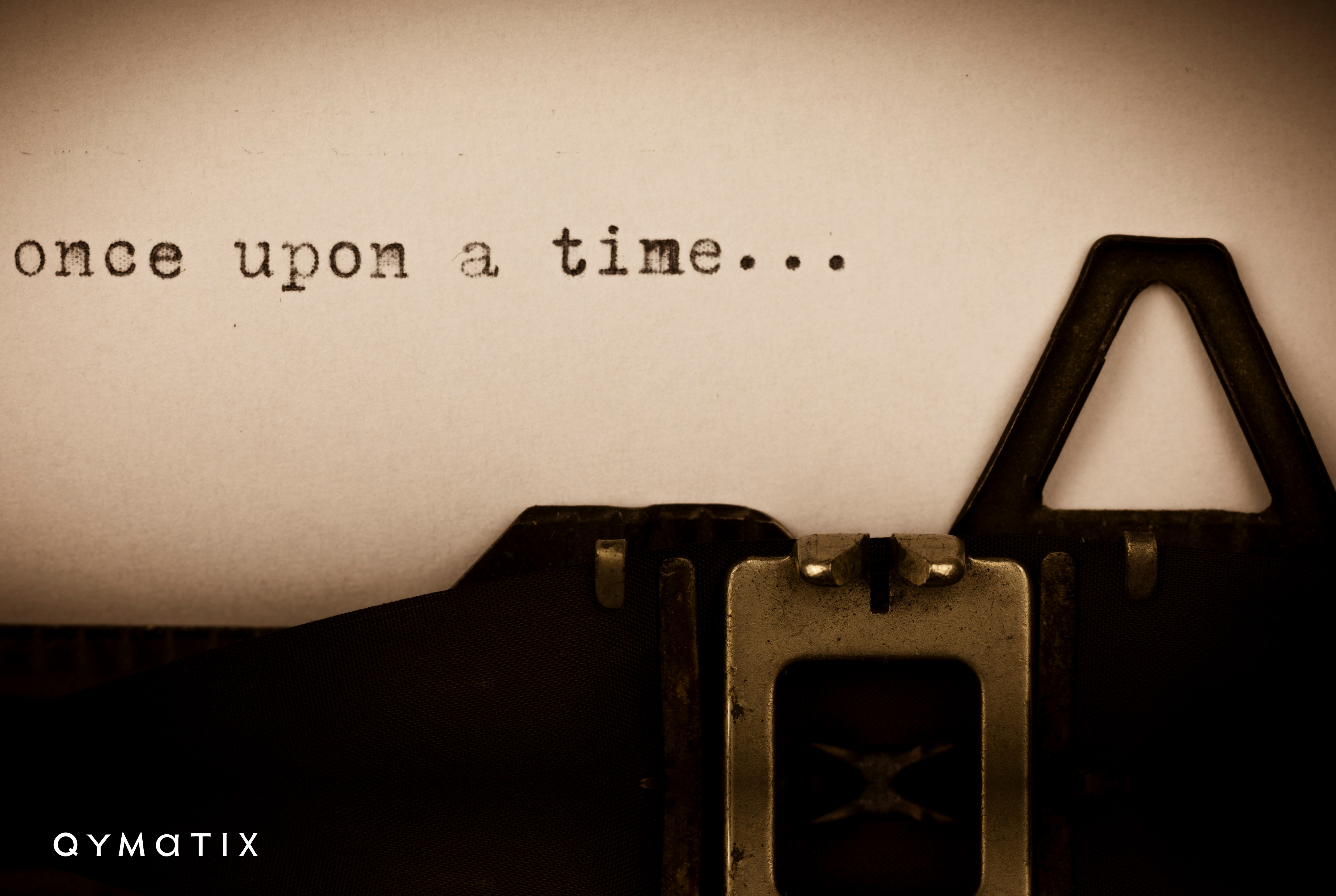
Please enter your Email address
This article outlines the key stages in the history of artificial intelligence (AI) and machine learning (ML) up to current applications.
You will also learn what role AI and ML play in today’s B2B business processes and why they are essential for predictive analytics.
The history of artificial intelligence (AI) and machine learning (ML) is marked by remarkable advances and groundbreaking discoveries. From the first theoretical concepts to today’s applications in various fields, AI has become a fascinating and unstoppable field.
This paper first examines the significant milestones and developments that have shaped these technologies. Then, in short sections, we highlight the revolutionary impact of AI and ML on the B2B sector and how they are transforming business. Finally, we look at the prominent role of AI and ML in predictive analytics and how they are helping companies make informed decisions, predict future events, and stay competitive.
The Origins of Artificial Intelligence
The roots of artificial intelligence go back to the 20th century when pioneers such as Alan Turing and John McCarthy began to explore the idea of machine intelligence. Turing developed the famous Turing Test to determine whether a machine could simulate human-like reasoning. McCarthy coined “artificial intelligence” and initiated the first AI conference in 1956.
Expert systems and the advent of machine learning
In the 1960s, expert systems were developed in which the knowledge of experts was stored in computers in the form of rules. That made it possible to automate specific tasks. In the 1980s, the age of machine learning began with the introduction of algorithms such as backpropagation for artificial neural networks. Backpropagation is an algorithm used in AI to improve the accuracy of the results of an artificial neural network. Backpropagation is a learning process that allows computers to learn from data and improve their performance. Backpropagation is a learning process that will enable computers to learn from data and improve their performance.
Neural Networks and Deep Learning
Neural networks boomed in the 1990s. Extensive networks of artificial neurons were developed to solve complex problems. However, the popularity of neural networks has only increased in recent years with the advent of deep learning. Deep learning allows computers to learn hierarchies of features and perform complex tasks such as image and speech recognition.
The emergence of chatbots and virtual assistants
As AI technologies have advanced, chatbots and virtual assistants have become more intelligent. They can now understand natural language and perform user-centric tasks. Chatbots are text-based dialogue systems that allow you to chat with a technical system. Companies are using them to improve customer service and answer customer questions efficiently. Who would have thought that we could talk to our devices and delegate tasks a few years ago?
Current applications and prospects
Today, we find applications of AI and ML in diverse fields such as medicine, robotics, autonomous driving, finance, and more. The technologies continue to evolve and are expected to have an even more significant impact. However, there are also discussions about ethical issues and the responsible use of AI.
The transformative role of Artificial Intelligence and Machine Learning in B2B
– Increasing efficiency through automation
AI and ML enable more efficient automation of business processes. Routine tasks such as data processing, reporting and inventory management can be automated, saving time and resources. For example, intelligent systems can use ML to analyze large amounts of data and provide relevant insights to make informed decisions.
– Personalization and improved customer experience
AI and ML are critical in personalizing offers and improving the customer experience in B2B. Companies can create customized offers by analyzing customer behaviour and preferences and providing them with a personalized shopping experience. Chatbots and virtual assistants powered by ML technologies also enable faster and more efficient customer communication.
– Prediction and preventive maintenance
Thanks to AI algorithms, companies can make accurate predictions and forecasts for their business models, described in more detail in the next section on predictive analytics. In addition, ML-based preventive maintenance enables early detection of failures or maintenance needs for machines and systems to minimize downtime.
– Security and fraud detection
Security plays a central role in B2B. AI and ML help detect threats and identify fraud patterns. By analyzing user behaviour and transaction data, suspicious activity can be detected early, and security measures can be taken. AI-based systems can also help protect networks and data from attacks and identify vulnerabilities.
– Business Intelligence and Decision Making
AI and ML technologies provide organizations with valuable insights into their data and support decision-making. Companies can identify patterns and trends by analyzing large amounts of data, performing competitive analysis, and making strategic decisions. By automating the processing and analysis of data, companies can respond more quickly to market changes and increase their competitiveness.
The breakthrough role of artificial intelligence and machine learning in predictive analytics
– Predicting customer behaviour and needs
The use of AI and ML in predictive analytics enables companies to predict the behaviour and needs of their customers. Companies can develop personalized offers and marketing campaigns by analyzing historical customer data. They can also anticipate potential customer churn and take action to retain customers.
– Risk mitigation and fraud detection
Predictive analytics based on AI and ML enables companies to identify risks and predict potential fraud: by analyzing historical data and identifying patterns, companies can create sales forecasts, predict demand, optimize production and storage capacity, identify potential problems early, and take appropriate action to minimize losses and reduce risk. That is especially important in the insurance, financial, and e-commerce industries.
– Optimization of business processes
AI and ML enable business process optimization through prediction and forecasting. Companies can use predictive analytics to anticipate production, supply chain, or customer service bottlenecks and take appropriate action to avoid them. This results in improved efficiency, cost reduction and customer satisfaction.
– Market analyses and competitive advantages
AI and ML allow businesses to conduct market analysis and gain a competitive advantage. Companies can identify opportunities early by analyzing market data and trends and adjust their strategies accordingly. Companies can make better decisions and strengthen their competitive position by understanding the market and customer needs.
CALCULATE NOW THE ROI OF QYMATIX PREDICTIVE SALES SOFTWARE
The Evolution of Artificial Intelligence and Machine Learning – Summary
The history of artificial intelligence and machine learning is an exciting journey through humanity’s efforts to expand the capabilities of computers. We have seen a remarkable and rapid evolution from the first theoretical concepts to today’s intelligent systems.
Artificial intelligence and machine learning have also played a transformative role in B2B and predictive analytics. From automating processes to improving the customer experience, making informed decisions, predicting future events, and recognizing patterns in big data, these technologies enable risk mitigation and business process optimization.
Organizations leveraging predictive analytics based on AI and ML can gain a competitive advantage and improve performance in an increasingly data-driven world. Now, we can look forward to the future of AI and ML and how they will continue to impact our lives.
I WANT PREDICTIVE ANALYTICS FOR B2B SALES.
Further Read:
Clickworker (2022): Die Geschichte des maschinellen Lernens – eine Zeitreise