If Your Data Is Bad, Your Sales AI Tools Are Useless
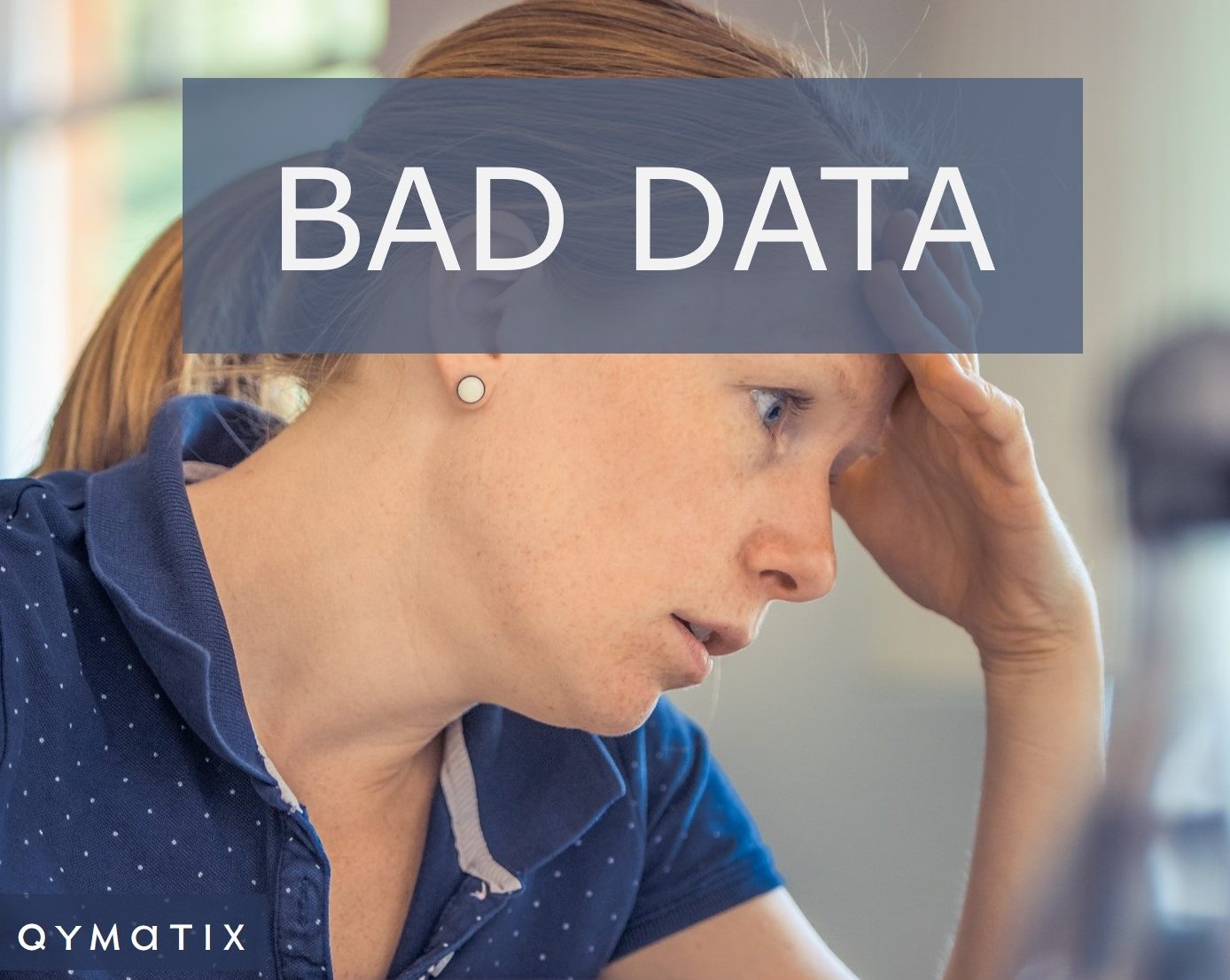
Artificial intelligence (AI) is steadily advancing in B2B sales. AI is changing the way customers are buying and therefore how salespeople should work.
The gathering of data, its quality, the source systems, all play a central role in the implementation of AI in Sales. Poor data quality may be hard to measure, but it takes an essential part in the application and execution of artificial intelligence systems and predictive analytics.
Regardless of the role data quality plays, companies should not wait long to start with AI in Sale. Executives ought to begin analysing their available sales data. No dataset is perfect. Once you have implemented an AI solution for sales, it is easier to identify where exactly to invest in data quality.
Lastly, the use of artificial intelligence in sales requires a continuous iteration and improvement on data quality.
Let’s review each concept in turn.
Artificial intelligence: What is the role of Data Quality in AI investments?
Data quality is critical in AI. Many of the most advanced sales AI techniques and machine learning (ML) algorithms require vast amounts of data. AI solutions for sales train these algorithms to discover new insights, such as churn risk or cross-selling opportunities.
For these advanced analytical methods, data quality is vital. Poor data quality in analytics diminishes performance. Often Business Intelligence and Big Data Analytics rely more on the quantity and quality of the sales data, than in the specific algorithm used to perform the analysis.
Data quality is medium to an end.
However, do not forget that data quality is medium to an end. In machine learning, one can assume that the analytical models are the boat and the data is the wind. Of course, you need a robust and reliable boat to sail, yet you still need the wind.
Moreover, there is no “good” or “bad” wind, no. Assessing data quality is not a binary operation. As in the sailing example, one will have very different categories of winds. In other words, the data alone does not determine how valuable the analytical results will be. You still need the right boat for the available wind – and an experienced crew and skipper.
Data quality is essential for artificial intelligence in sales as it is for any other machine learning application. However, managers should focus on how relevant the data is for the method or algorithm that they are training.
How can you determine if the quality if your data is good enough for analysis that you are trying to perform? The best answer is to estimate the ROI (Return on investment) of the entire model, considering for weaknesses in data quality and possible needed adjustments.
AI in Sales: Get started with the data that you already have.
The ideas above bring us to an essential topic in AI sales. No data set is perfect. Do not wait to get started until you have complete and precise data, for you will wait a long time.
Considering the wind-boat example again, if you expect for perfect conditions to navigate, you will mostly remain on the dock.
What you can do is to get started with the data that you have (ERP, CRM), while focusing on specific data quality aspects that are relevant for your data analysis model.
For example, in a recent implementation project of our Predictive Analytics Software, the customer provided us with three data sets: ERP sales transactions, CRM activities and a database of their product portfolio.
No data set is perfect.
The ERP dataset had around 10.000 sales transactions per month with approximately 500 customers. There were 3.000 CRM activities, including account information about customers. Finally, there were 2.300 products in the product database, aggregated in almost 30 product groups.
Was data quality “good”? Yes and no. In this project, we were applying our software to discover cross-selling potential. Some specific aspects of data quality were, therefore, highly relevant to the predictive model.
ERP sales transactions had some flaws, mainly due to formatting. Flaws in format is something an algorithm can consider. CRM data was good enough – addresses missing, for example.
However, a critical issue was the missing product group in around 15 % of the total articles. This aspect of the data quality had a real impact on the performance of the model.
I want to talk with you about my sales data.
Artificial Intelligence & Data Quality: Improve slowly and invest wisely
Following on the example above, from several data quality aspects, only one was relevant to the kind of data analysis our predictive sales software was trying to perform.
We worked together with our customer to estimate the impact of this specific aspect of data quality and suggested some corrective actions. Once we had our first models up and running, the customer improved the product data set and instructed his team to avoid adding new products without a given product family.
Data science done right is always an iterative process. The example we presented above recaps the minimal three steps you will to fruitfully implement a Sales AI project: start with the data that you have, check for opportunities to improve the model based on data quality and invest there where necessary.
Investments in data quality can have different tenors, for measuring and defining data quality is not a crystal-clear matter. There are at least four aspects related to data quality, and you might have to invest in one or more.
The quality of the sales data available for your artificial intelligence tool can be defined by your access to information (system access, security, stability), data representation (interpretability, comprehensibility, manipulability, integrity and freedom from contradiction), the information context (relevance, additional benefits, topicality, completeness, scope of information) and its intrinsic value (correctness, objectivity, credibility, reputation).
Once your Sales AI tool is presenting its first results, define if there are data quality aspects mentioned above that might need further investment. Invest only where you have a clear case and an estimated high ROI.
CALCULATE NOW THE ROI OF QYMATIX PREDICTIVE SALES SOFTWARE
If Your Data Is Bad, Your Sales AI Tools Are Useless – Summary
The use of artificial intelligence in sales strongly relies on data quality. If data quality is suboptimal, a company will not harvest the full benefits of an AI solution.
Companies should take actions to measure and improve data quality. Moreover, where precisely to invest, should be defined by the AI analytical methods. For example, AI predictive pricing needs investment in ERP data quality, whereas Lead Scoring might need improvements in CRM data quality.
Companies should not wait until they have addressed data quality issues to begin using AI in sales. The output of the AI system will narrow down the necessary investments in data quality. This iteration will improve both the data and the AI solution.
AI in sales requires a continuous evaluation of data quality. Executives should see AI systems as evolving entities to which improvements in data quality will convey better outputs and higher ROIs.
Artificial intelligence (AI) is going mainstream in sales and defining data quality needs. Contact us for a more in-depth discussion of your data needs.
Free eBook for download: How To Get Started With Predictive Sales Analytics – Methods, data and practical ideas
Predictive analytics is the technology that enables a look into the future. What data do you need? How do you get started with predictive analytics? What methods can you use?
Download the free eBook now.
- We will use this data only to contact you for discussing predictive sales KPIs. You can read here our declaration on data protection.
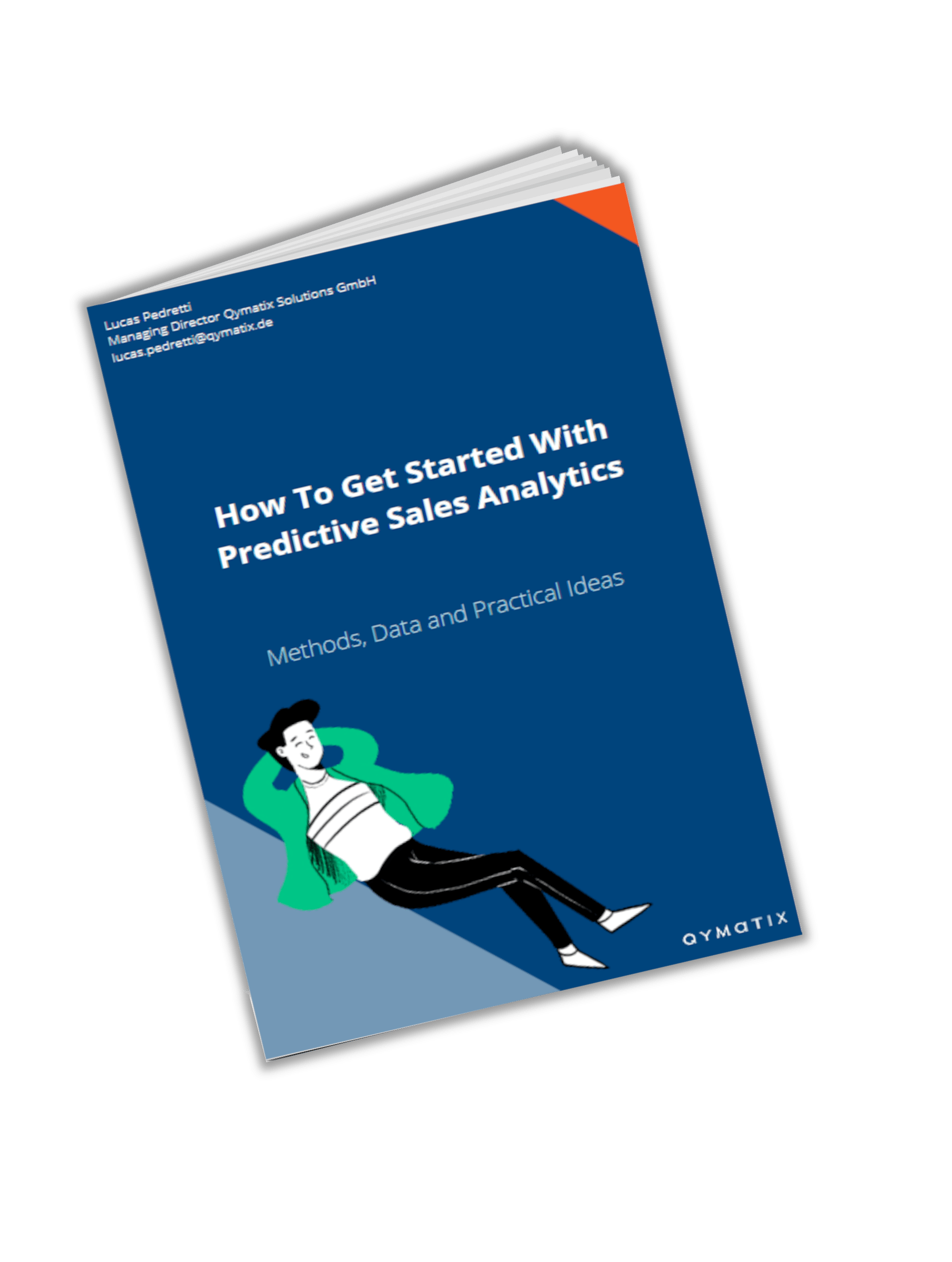
Further Read:
Victor Antonio (2018). “How AI Is Changing Sales” – Harvard Business Review