Forecasting Individual Customer Lifetime Value: Why You Should Not Use External Data
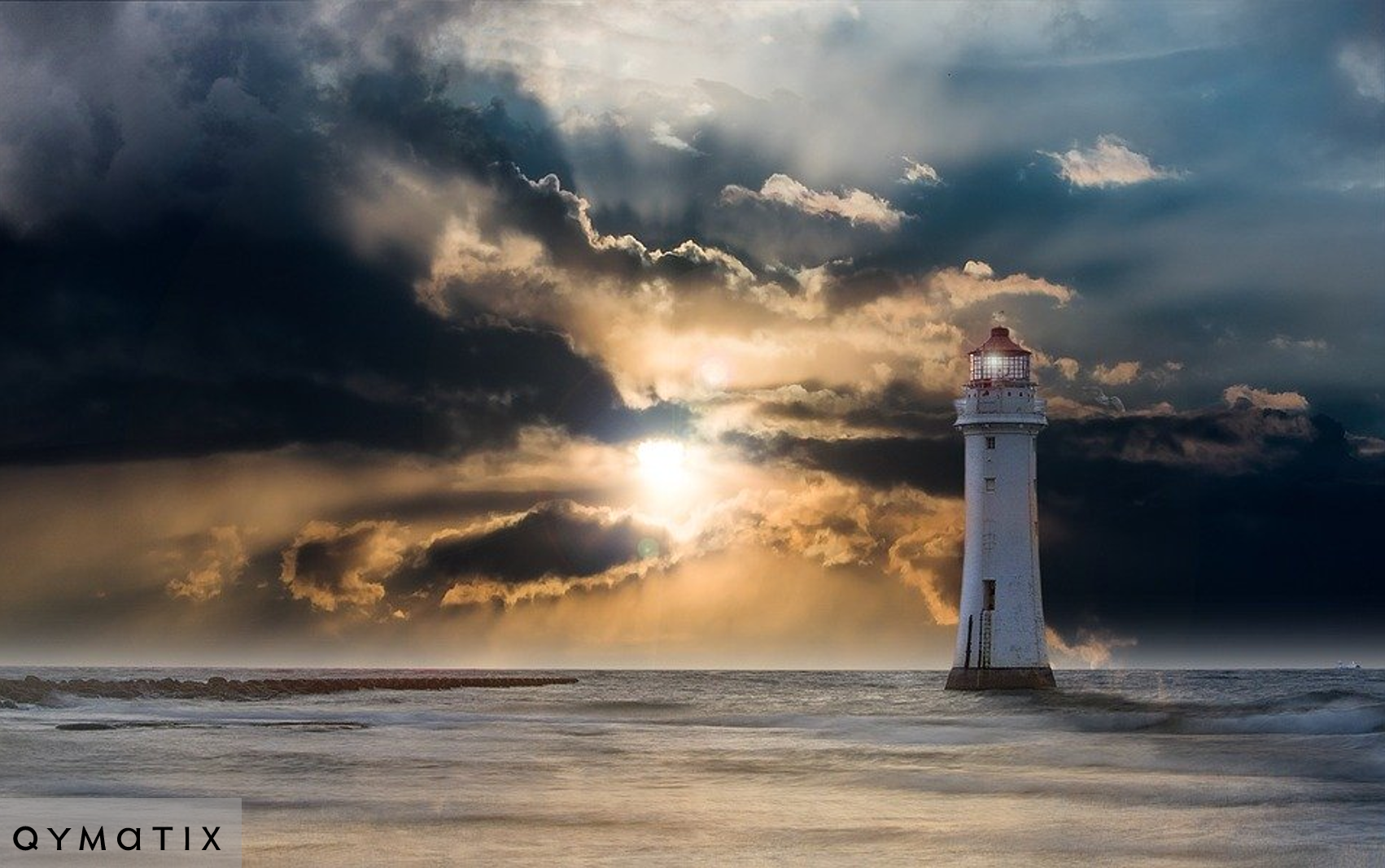
There are two types of historical data to use for predictive analytics and sales forecasting: internal and external data. How to know which one to choose?
Making a forecast always requires planning under conditions of uncertainty. Successful sales executives plan and execute an accurate sales forecast using data. For an aggregated, precise sales plan, it is necessary to consider forecasting the individual customer lifetime of the client base.
There are different data sources that executives can use to forecast sales. Some of them count for internal factors while others count for external factors.
The former relates to changes in supply-side, product lines, the sales team, or sales commissions, among others; the later relates to competitive movements, industry changes, market changes and general economic conditions.
While it is tempting and relatively easy to do, sales managers should avoid using external data to predict or forecast the individual customer lifetime. By external data, we are referring to the anticipated gross domestic product or industry-wide predictions, even customer surveys about demand.
Although the general data quality of external data sources can be good enough, external data sources are incredibly inaccurate to create sales forecasts.
Fortunately, B2B companies possess more consistent, robust and economic data sources to forecast sales individual customer lifetime: internal ERP sales data and CRM data. Let’s discuss each point in turn and see how can you avoid from missing on your sales forecasts.
How did Executives Forecast Sales and Individual Customer Lifetime in the Past?
Nobody has a glass-ball. Sales executives and upper management still need to create reliable sales plans and to forecast sales figures, aggregated, per product, and customer. Sometimes yearly, quarterly, or over a multi-period. Predicting each customer lifetime is the Everest of sales forecasting, is possible, nonetheless.
To overcome this challenge, sales practitioners in B2B have been employing a series of forecasting methods, using different tools, on various data sources.
Predictive analytics is the umbrella-name encompassing sales forecasting methods. Is it better to use a data mining tool or management software? Most sales managers are still getting busy with powerful Excel to perform and operationalize predictive forecasting. Nevertheless, 86 % of companies in a recent survey answered employing a BI solution for data consolidation, planning and forecasting.
Today we focus on the question: “What kind of data can a sales manager use for sales forecasting?” As already mentioned, there are many types of data sources for this purpose. One thing is often misunderstood: just because a sales forecast is an internal factor does not automatically mean that only internal data sources may be employed. Internal factors can be predicted with external data and vice-versa.
Internal versus External Factors for Forecasting Individual CLV
Sales forecasting is not an exact science. It is the discipline of uncertainty and complexity. To reduce complexity, we can split the factors that affect sales forecast into internal or external factors.
Internal factors can be related to changes in the sales team, in production, supply-side, product lines, and changes related to sales commissions, among others.
External factors can be related to competitive changes, industry changes, market changes and general economic conditions. This last factor is usually present in conventional sales forecasts, for example, as the gross domestic product (GDP) of the country or region where the clients are based.
There is a strong rationale to link economic conditions to customer’s demand. If the general economy is stable, customers are more likely to invest in their businesses and to experience rising demand themselves. If the economy is weak or in recession, the sales cycle will take longer; revenues might stagnate or go in free-fall.
In summary, the expected general status of the economy is usually considered a safer anchor for a sales forecast. If, for example, the GDP of India is going up 5 % next year, a sales manager could forecast a similar level of increment on her revenues.
Is it, nonetheless, a good idea? Will it produce an accurate sales forecast? What impact does the predicted GDP of a region or country into the individual customer value?
Whether internal or external factors have a higher impact on a sales forecast is only partially a topic of discussion. Today we are arguing about what kind of data is available and whether it is accurate enough and inexpensive to gather.
How do Sales Leaders Forecast Individual Customer Lifetime, and What Data Can They Use?
How can you predict CLV? The first KPI you can calculate is the historical CLV. Executives calculate the lifetime value of an individual customer by adding all previous profit assigned to it. Predictive CLV is the future sum of the historical CLV.
Many sales managers employ external data, such as GDP levels, in their predictive CLV calculations. However, how good are these external sources of data for forecasting customer lifetime?
Using external data sources to forecast individual customer lifetime presents at least two difficult challenges. First, what kind of external data can you use? Second, how reliable is that data?
Selecting external data is critical. Unless you can ask every customer how much they are planning to buy from you, other external data sources will not correlate very weakly with your revenue. Furthermore, even if you do ask your customers about their future demand, they might fail to fulfil their orders.
How Accurate and Reliable are External Sources of Data for Forecasting Individual Customer Lifetime?
External data sources are extremely unreliable and inaccurate. Imagine you want to assess the impact of the general economy or the GDP in your future revenues, would you use predictions of the IMF (International Monetary Fund) for your forecast?
Using, for instance, IMF data for forecasting individual customer lifetime is a terrible idea. A recent 3,200 same-year country forecasts analysis conducted by the information company Bloomberg found a significant disparity in the magnitude and direction of errors. In more than 94 % of the cases, the IMF missed GDP growth, with a small tendency to underestimate it.
The average IMF forecast is wrong by 2.0 percentage points. Regional differences? Yes, the IMF overestimated the U.S. growth four of five times while China’s growth only once every five.
The trouble is not only with forecasting models but also with hard data. Economic data are adjusted continuously, even in countries with reliable data. For example, your ERP sales data is much more reliable here.
Use internal data sources to produce more accurate sales forecasts.
There are more reliable, trustworthy and inexpensive data sources to forecast sales individual customer lifetime: internal ERP sales data and CRM data. The main logic behind using these internal datasets is that they are closely related to your target variable: customer lifetime value.
For example, what are the three key factors influencing CLTV? Sales potential (in the form of cross- and up-selling), churn, and pricing. The price alone has the highest impact on earnings and the churn in the longevity of the customer relationship.
However, not only pricing and churn have an impact on future CLTV. All things equal, realizing cross-selling opportunities also have a remarkable effect on future CLTV. Otherwise, simply assume that the customer will keep on buying the same – a sort of basic linear regression.
Other Inexpensive, Internal Data Sources Available for Forecasting Individual Customer Lifetime
There are further internal data sources a sales executive can use to successfully generate more accurate sales forecasts. Among some of them, we can list product-generated data, web-shop and website interactions, and customer service (complaints and dealings with after-sales teams).
For product generated data, imagine, for instance, a machine reading usage via its sensors, a credit card, a car, an electrical installation. They all produce data that executives can use for their sales forecast.
Web-shop interactions, behind what actual sales remain in the ERP system, can also be an interesting data source. What are customers searching? How is the number of interactions in the shop evolving? How does the hit-rate in the shop compare with off-line sales?
Lastly, since many customers’ journeys begin with online interaction, web activity such as the number of visitors, bounce rate, and pages per visit could be integrated into the sales forecast. It is, nevertheless, hard to correlate anonymous online activity to specific customers. This data source might, therefore, not be very useful to forecasting individual customer lifetime value.
In summary, there are many available, reliable and inexpensive internal sources of data for predictive analytics and sales forecasting a sales executive can use. With increasing global competition, signs of a recession, and more knowledgeable customers, companies cannot miss the opportunity to generate accurate sales forecasting.
CALCULATE NOW THE ROI OF QYMATIX PREDICTIVE SALES SOFTWARE
Forecasting Individual Customer Lifetime: Why You Should Not Use External Data – Summary.
“Sales plans are nothing; forecasting individual customer lifetime is everything.” Or so it goes the B2B sales folklore. If you are not looking into the future of your sales relationships, you are missing on insights and money.
To predict total revenue, a sales executive can aggregate the forecasting of individual customer lifetimes. Forecasting sales involves planning under uncertainty – full of known unknowns. There are, however, logical ways to reduce uncertainty. One way is building a sales forecast with reliable, first-hand, data – no with unreliable, second-hand, predictions.
Do not expect to get a reliable sales forecast from external sources of data, especially not from predictions related to general economic conditions.
Which data sources do you use for sales for forecasting individual customer lifetime? Comment below!
I WANT TO USE PREDICTIVE SALES ANALYTICS TO INCREASE THE CLV
Free eBook for download: How To Get Started With Predictive Sales Analytics – Methods, data and practical ideas
Predictive analytics is the technology that enables a look into the future. What data do you need? How do you get started with predictive analytics? What methods can you use?
Download the free eBook now.
- We will use this data only to contact you for discussing predictive sales KPIs. You can read here our declaration on data protection.

Further Read:
Economic Forecasting Is Really Difficult. Just Ask the IMF. Ed.: Bloomberg
IMF shows poor track record at forecasting recessions. Ed.: Financial Times
Michael P. Clements: “An Evaluation of the Survey of Professional Forecasters Probability Distribution of Expected
Inflation and Output Growth” Ed. Journal of Economic Literature, November 22, 2002.
Pufahl, M. (2018): Sales Performance Management. Ed.: SpringerGabler