Predictive Customer Analytics: From customer analysis to customer value
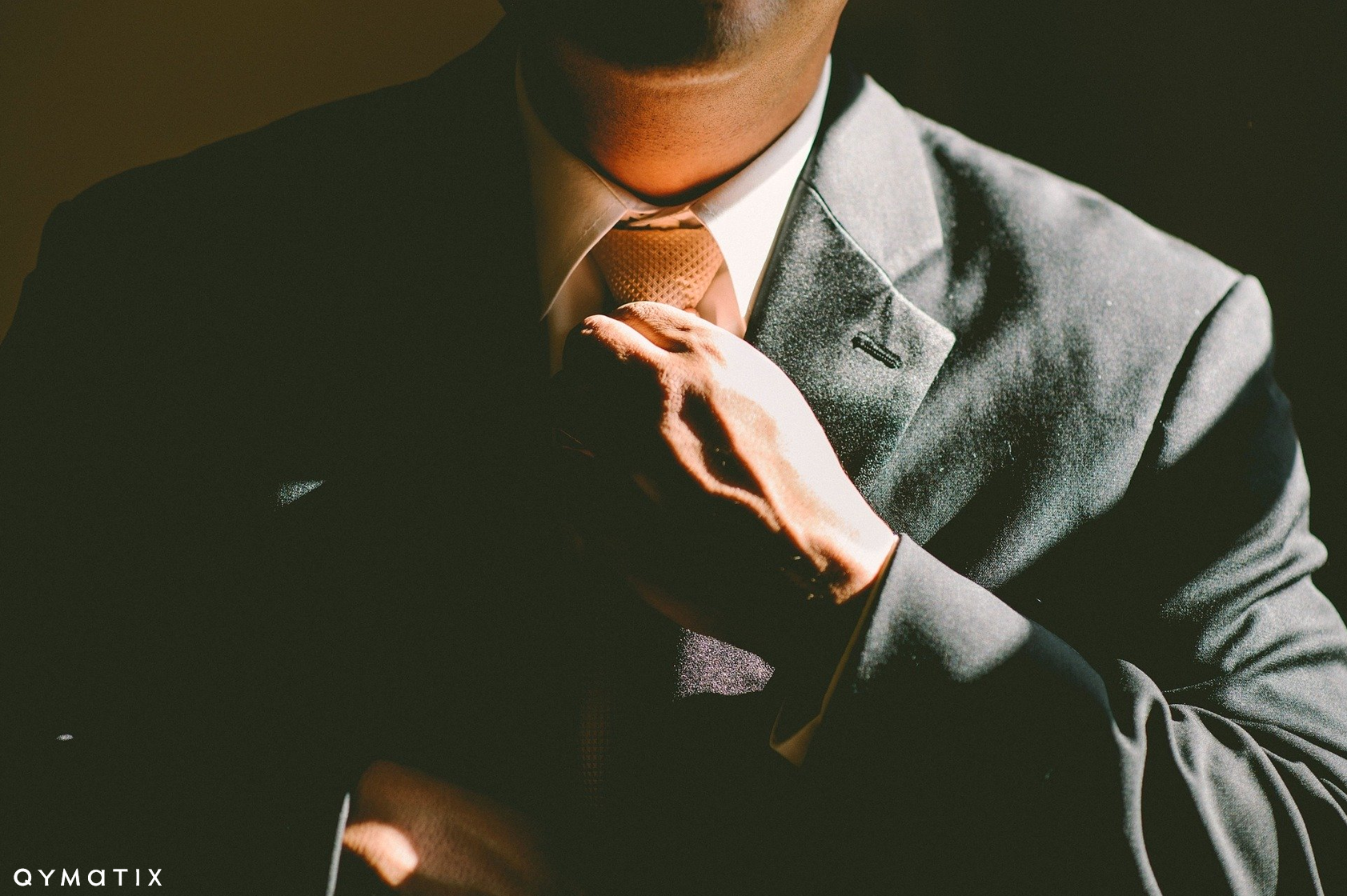
Customer analytics with predictive analytics can provide valuable information for sales activities.
Do you really know your customers – and not only the large-scale customers? Successful Customer Analytics should provide you with all the critical information about your customers. What are their preferences? How do they shop?
Using customer data, predictive analytics can determine individual opportunities for offers and subsequently determine the value of a customer.
The goal of customer analytics is to learn more about customers. The better companies know and understand their customers, the better they can adapt their services to their needs. Customer Analytics provides answers to the following questions:
– How does the customer structure currently look?
– What do my customers need?
– What do my customers expect from me?
– How much does a particular customer or customer group bring me?
Customer data as the starting point for customer analysis
Today, companies have masses of customer data. Especially companies that operate on several channels and address their customers there (multi-channel) can access data and data traces (big data). The preferences and buying behaviour of the customers can be analyzed.
Sources for this can be an online shop, social media or simply sales transactions. From this data, you can derive patterns and correlations. And predictive analytics can make forecasts about future customer behaviour.
The customer structure can be determined using different characteristics. You can evaluate the following examples of criteria:
– product portfolio: What products do customers buy?
– Seasonality: Times of purchase.
– Regularity: At what intervals do customers buy?
– Sales development: Sales per customer and product.
– Information behaviour: Communication behaviour, media use.
– Purchasing behaviour: Brand choice, brand loyalty, price awareness, value of the average purchase, frequency of new purchases.
– Usage behaviour: Intensity, type, environment, recommendation behaviour.
– Geographic criteria: National territory, urban areas, residential areas, city, country.
– Demographic criteria: Age, sex, marital status, number of children, household size (rather only in B2C).
– Sociographic criteria: Income, purchasing power, level of education, occupation, ownership characteristics (rather only in B2C).
– Psychographic criteria: Motives, attitudes, benefit expectations, personality traits, preferences (rather for the B2C sector only).
With the help of these criteria, companies can determine the structure of their typical customers in great detail. However, you should also make sure that you make a comparison with the top customers since they account for a large part of sales and may differ considerably from the typical customer.
From customer analysis to customer value
Basically, customer needs are wishes or problems that customers want to have fulfilled or solved.
In the B2B sector, these are the company goals, such as growth, increased sales or a good competitive position. However, customer expectations are often individual and relate to specific services or products. These expectations are constantly changing and should therefore be determined on an ongoing basis.
If the expectations or needs of the customers are very different, you should make a differentiation between the customer groups. For example, it is possible that some top customers are satisfied with certain products or services while others are not, but they tend to generate small sales.
That leads to the next important point of customer analysis: The actual value of a customer for the company – the customer value.
Customer value is about how much an individual customer or group of customers contributes to the economic success of the company.
That can be determined by analyzing customer data using predictive analytics.
The customers who make a significant contribution to economic progress are the key accounts. In sales, the key accounts often receive individual support.
However, you should not only determine customer value by turnover. Besides the purely monetary customer profitability, the information and reference value of a customer also plays a role. Sales or contribution margin per customer determine the monetary value. Contrary to this, there is the information value. It consists of all the information provided by customers and used by the company.
These could be, for example, customers who are an important reference or even customer complaints which stimulate improvements and performance increases in the company.
I want to use predictive customer analytics today.
Methods for determining customer value
Conventional methods for determining customer value are:
– ABC Analysis
You divide customers into categories A, B and C, each according to their share of total turnover. That shows where targeted measures are worthwhile or where detailed budgeting is required.
– Customer contribution margin calculation
It is about the contribution margin of a particular customer after all costs. However, only deduct the expenses that you can assign to this customer.
– Customer potential analysis
A customer potential analysis evaluates the future chances of individual customers to gain indications for the differentiated processing of these customers. Modern software for predictive analytics makes this easier.
– Customer portfolio analysis
Concerning customer value, customer portfolio analysis means that the funds invested in the respective customer must be in a balanced relationship to its profit potential.
– Customer Lifetime Value (CLTV)
To calculate the Customer Lifetime Value, you estimate the profitability of a customer in the form of its capital value for specific periods of the business relationship. Thus, you consider the “lifetime” of a customer. That applies to every type of business relationship, including B2B customer lifetime value.
– Scoring Model
In the scoring model, all transactions with a customer are weighted and given positive or negative points. You use the weighted points value for customer classification.
Methods such as ABC analysis or customer contribution margin accounting only refer to the past.
The main focus is on analyzing what individual customers have achieved, not what sales potential or sales opportunities they might still have. That is precisely where predictive analytics comes into its own:
As a subset of Business Intelligence (BI) and Business Analytics (BA), Predictive Analytics starts where Online Analytical Processing (OLAP) or Reporting ends. It not only analyzes an existing customer situation but also attempts to use data models to make predictions about possible future events.
That can be the potential for cross-selling and up-selling, or even a possible customer churn risk. With predictive analytics, companies look forward, so to speak, and not just backwards. They make data-based decisions.
CALCULATE NOW THE ROI OF QYMATIX PREDICTIVE SALES SOFTWARE
Predictive Analytics prepares the determination of customer value.
Whoever determines the monetary customer value should take into account that the customer’s income value also depends on his payment behaviour. It is therefore crucial whether the customer makes the payments in the agreed amount and time.
In this respect, predictive analytics not only provides insights into customer behaviour but also makes it possible to forecast future customer activities.
Customer analysis with predictive analytics should considers the customer journey.
So, it can provide valuable information for decision-making and a suitable selection of targeted sales activities. The data obtained is an important source of information for several business units: marketing, sales, product development and other decision-makers.
In conclusion, customer analysis provides companies with valuable information about the structure, needs, expectations and behaviour of their customers. Furthermore, it determines their actual value to the company. These are essential fundamentals from which measures to improve the performance of the company can be derived.
How relevant is the topic of customer analytics to you? Contact us!
I want to start today using predictive customer analytics.
Free eBook for download: How To Get Started With Predictive Sales Analytics – Methods, data and practical ideas
Predictive analytics is the technology that enables a look into the future. What data do you need? How do you get started with predictive analytics? What methods can you use?
Download the free eBook now.
- We will use this data only to contact you for discussing predictive sales KPIs. You can read here our declaration on data protection.
