Why is internal data considered more reliable and easier to collect than external data?
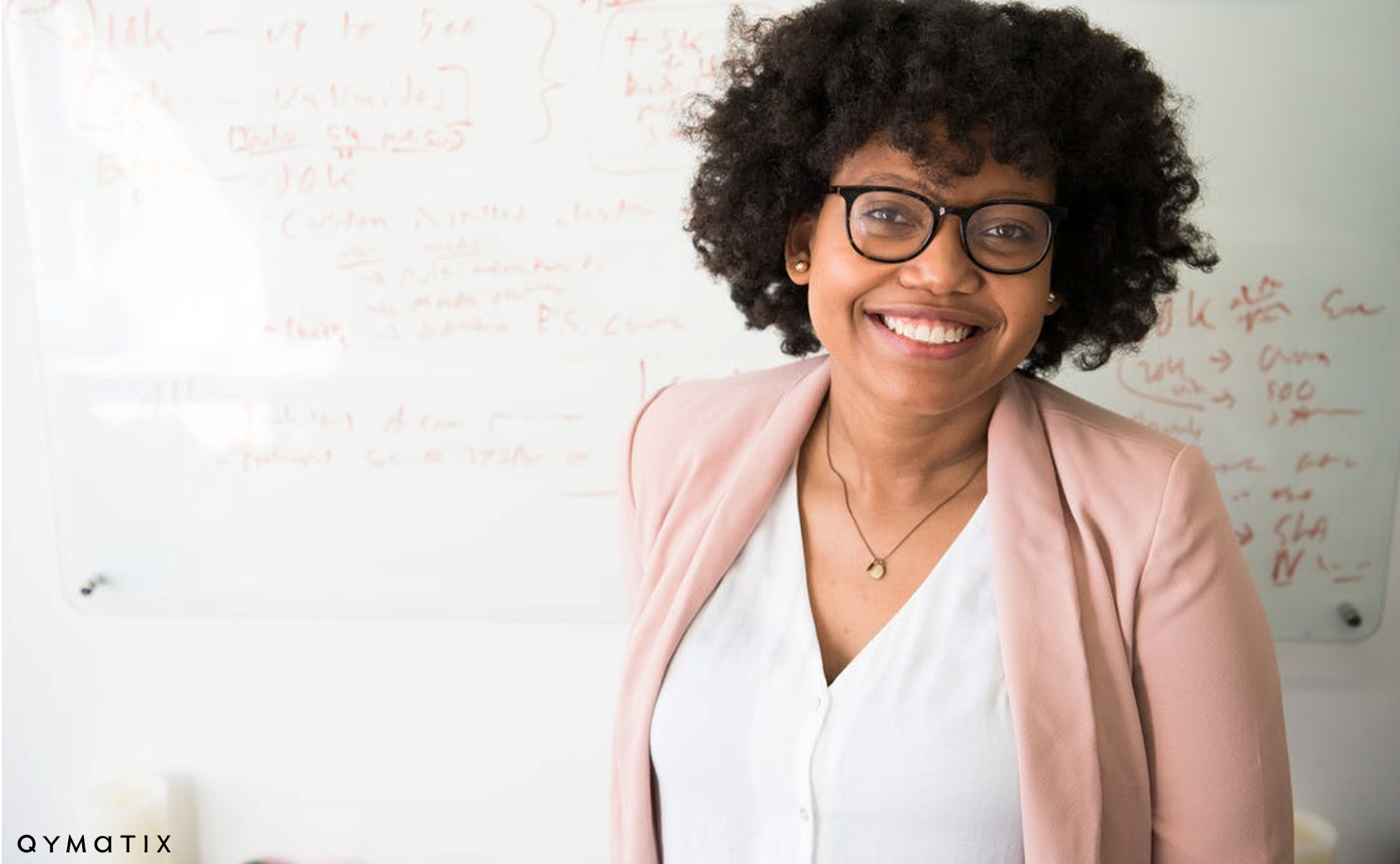
Simply explained: Why internal data is better for predictive analytics in B2B.
Companies use sales forecast to make business decisions. They also employ them to predict future developments better than their competitors. However, reliable predictions are rare, and sales teams try to play a safe card by applying external forecasts. Companies are nevertheless better off using their in-house data – with predictive analytics.
“There are three types of lies: lies, damn lies, and statistics.” This quote from Benjamin Disraeli, a British statesman and 19th-century novelist, fits the situation in companies very well.
They collect figures on sales, profits, losses, customer growth and decreases and much more – and generate statistics from them. A lot of economic data is collected and processed to flow into forecasts for the near or distant future.
Sales forecasts, for example, serve to adjust your individual investment decisions. In general, predictions are also used to foretell (possible) future developments better than the market.
Forecasts using predictive analytics models are generally superior to decisions based on gut instinct or human-intuition, even when it is clear that forecasts cannot be 100 per cent correct.
The problem of economic forecasts
International companies base internal company forecasts, i.g. on sales or profit development, on data originating from foreign markets. For example, globally active sporting goods manufacturers such as Adidas, Nike or Puma track economic developments in Asian markets, sometimes different from those in Europe or the USA.
Those manufacturers and its distributers who operate internationally and have customers in many countries also have an eye on the general economic situation and the expected economic development in these countries.
Forecasts on the economy, such as those prepared by major economic institutes or associations such as the Centre for European Economic Research (ZEW) or the Ifo Institute, are used by companies to support their own decisions for the future. By taking such – external – forecasts into account, company management can demonstrate farsightedness to employees and other stakeholders. However, economic Predictions also show that the underlying assumptions about the world and the future are not always correct.
The problems begin with the measurement of the current economic conditions. After all, governments and institutions need first to collect and process relevant data about the economy. Collection of financial data occurs only with a significant time-delay, sometimes of several months. Only then can economists make predictions and forecasts about the economy.
The more data available, the more frequently analysts revise estimates for past years.
Economic forecasts can therefore, at best make statements about short-term trends using long-term old data.
Complex, random events easy to forecast, but hard to predict.
Economic forecasts usually cover a time horizon of no more than two years. Any estimates beyond this are long-term forecasts of growth and general structural developments – and these are mostly extreme uncertain and full with randomness.
More problematic because we face a massive amount of data, sometimes helpful, sometimes noise. Even if cause-effect relationships are assumed, these are interwoven and in turn dependent on other effects and causes.
Let us take the current political disputes and the back and forth of Brexit. Or global trade conflicts, as we are currently experiencing between China and the USA. Economic forecasts are often inaccurate because there are too many factors and interactions involved.
In economic theory, there are connections among hundreds of variables. It’s about people’s behaviour, and one action depends on the other. If the current US President, Donald Trump, puts off a corresponding tweet, this could lead to significant economic irritations in individual markets tomorrow, if not in the global market.
Forecasts on the economic development of a country are also very quickly invalidated if unforeseen events occur, such as the bursting of the real estate bubble in the USA. Or an unexpected natural disaster or political unrest in an important export country. All these unforeseen events mean that forecasts are erroneous.
Using your internal data for predictive analytics
If economic forecasts are poor – or if their accuracy is unreliable due to the uncertainty factors we mentioned, – what other data can companies should rely on?
After all, companies need to predict complex economic relationships to make better decisions and gain a competitive advantage.
The answer may be trivial, but it’s still apt: companies should look at their internal company data. Predictive Analytics uses data models to predict how a situation will or can develop in the future. Predictive analytics is a sub-discipline of business analytics and starts where business intelligence (BI) ends. Instead of just analyzing the existing situation, Predictive Analytics uses data models to predict probable events in the future.
Data science makes predictive analytics possible.
For example, those who already know today what their customers will buy tomorrow have a clear advantage over the competition. With predictive sales analytics, companies can make such forecasts using well-tested forecast models.
One of the underlying assumptions here is that the more we know about the past (customer) behaviour, the more accurate we can make statements about future events.
Although such forecasts are possible scenarios that might occur with a certain probability, predictive analytics using internal data considers a set of robust assumptions: customers and vendors will behave in the future similarly as they did in the past.
Once the sales forecasts use meaningful data; for example, past ERP sales data, companies can significantly improve the quality of their business decisions. A central goal of Data Science is: To produce reliable forecasts about future events as part of predictive analytics.
Companies can use predictive analytics to gain new insights – a new understanding of their database and thus, information that may have remained hidden from them until now.
They use their internal data from the CRM or ERP. Based on this data on customers, portfolios, risks or price developments, for example, companies can derive statements that sustainably improve future-oriented decisions.
CALCULATE NOW THE ROI OF QYMATIX PREDICTIVE SALES SOFTWARE
Simply explained: Why internal data is better for predictive analytics – Summary.
Place your trust in forecasts from your company internal data, rather than sophisticated external economic forecasts.
Predictions from external data are highly dependent on external factors and subject to many uncertainties. Don’t get us wrong; we don’t want to say that you should completely forget your outside world – of course not, that would be fatal. But before you make your decisions based on all external sources, take a look at your internal data.
With predictive analytics, you can get the most out of your internal sales data. You would be amazed at what predictive analytics can predict about your customers’ buying behaviour: Future customer attrition risks, pricing leeway, cross-selling potentials, customers with high purchase probability and much more. All you need is what you already have: Your own ERP and CRM data.
In our opinion, only one question remains unanswered: When do you start?
CONTACT US TODAY FOR YOUR PERSONAL CALL
Free eBook for download: How To Get Started With Predictive Sales Analytics – Methods, data and practical ideas
Predictive analytics is the technology that enables a look into the future. What data do you need? How do you get started with predictive analytics? What methods can you use?
Download the free eBook now.
- We will use this data only to contact you for discussing predictive sales KPIs. You can read here our declaration on data protection.
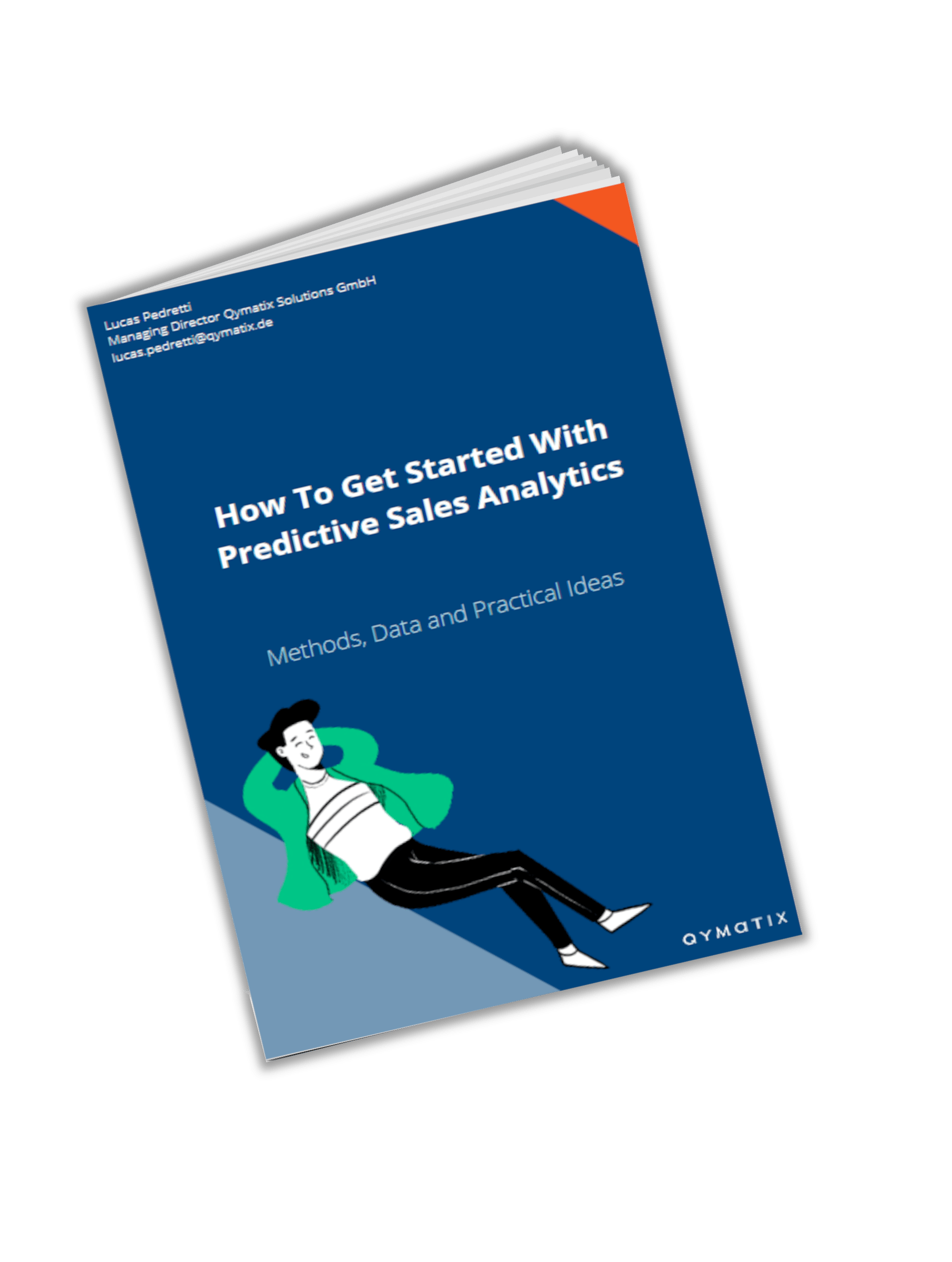