AI in B2B Sales? Machine Learning vs. Humans
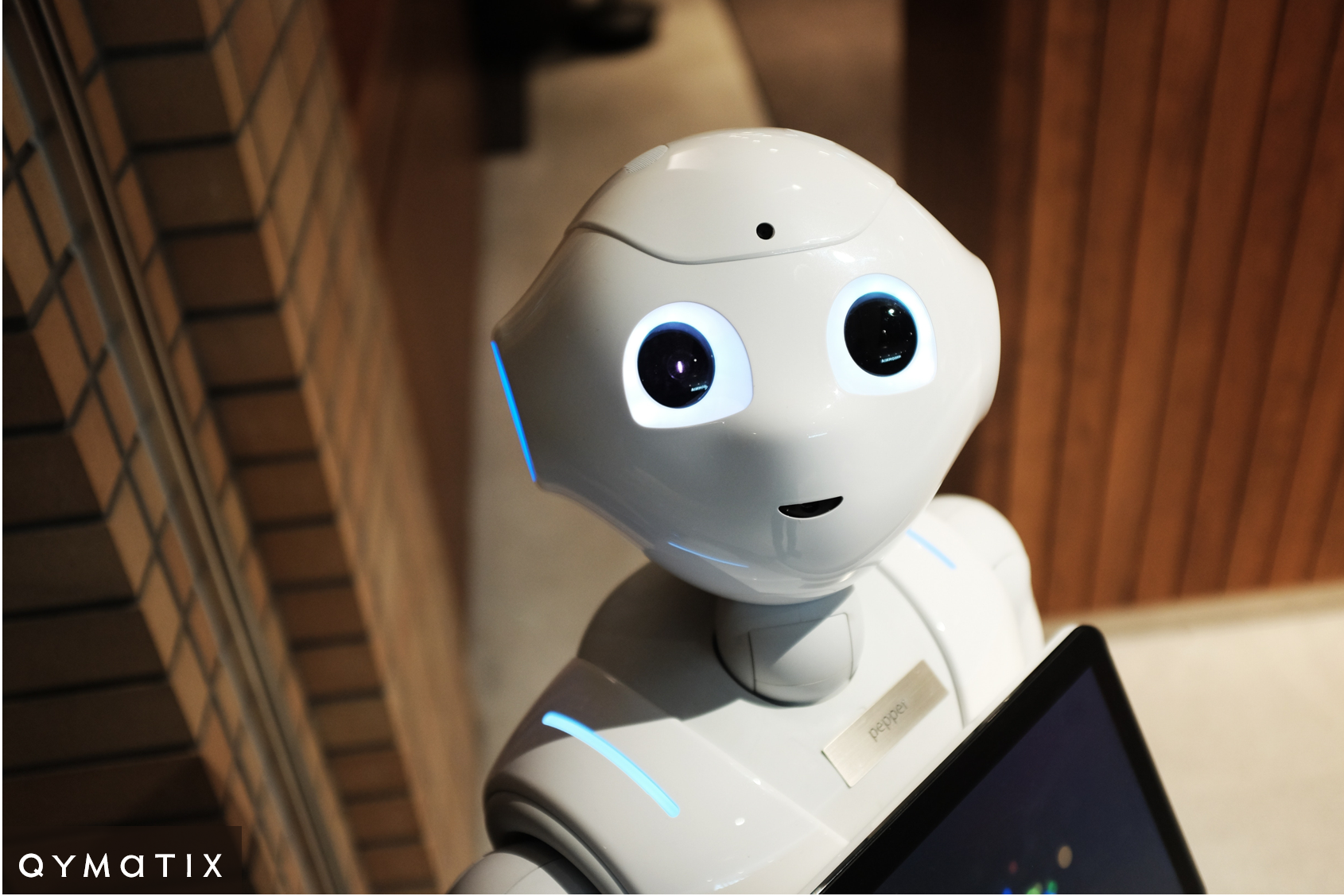
The interaction between humans and AI in B2B sales.
AI in sales uses machine learning to supply humans with sales insights and recommendations. Machine Learning generates these sales alerts using recurring patterns of customer data.
However, where direct contact between seller and buyer occurs, the human factor still plays a fundamental role. The automation of sales processes significantly reduces administrative tasks in a company. Algorithms replace individual manual functions that are performed by human hands and make our efforts redundant.
For example, companies are relieved of bureaucratic ballast and can concentrate fully on their core business. That also applies to B2B sales, of course.
The keywords here are also Machine Learning (ML) and Artificial Intelligence (AI). As early as 2018, Accenture chief technologist and chief digital officer Paul Daugherty described artificial intelligence as the fastest growing technology in the history of his company.
What had happened since then? What is Machine Learning in Sales, and why does it need humans to succeed.
What is Machine Learning?
What does AI in general, and Machine Learning in particular, mean for a company’s sales force nowadays?
Machine Learning is a sub-area of Artificial Intelligence. IT systems recognize specific patterns and regularities from existing data sets and algorithms. These findings can be generalized and used for new solutions.
Experiential learning happens on the artificial level because it is not a human being who experiences. In this respect, machine learning generates artificial knowledge.
Where can Machine Learning support sales teams in a way that not only makes processes more efficient and saves time but also increases sales productivity?
In short: by increasing the number and quality of available sales opportunities.
According to a study by the US market research company Cirrus Insight, average sales representatives spend only two days a week selling and contacting customers. Reps thus spend almost two-thirds of their time on administrative tasks such as lead qualification, travel or internal meetings.
Machine learning is unthinkable without humans.
Concerning sales, you can use machine learning to identify specific patterns from a data stock (like a CRM- or ERP-System). For example, an ML solution can classify and prioritize sales leads based on their buying-likelihood or can recognize which customers have a higher risk of churn. IT systems that rely on machine learning can
– Find, extract and summarize relevant data
– make predictions based on the analyzed data
– calculate probabilities for certain events
– Optimize processes based on the recognized patterns
However, a human being must first determine the underlying rules and algorithms that perform these arithmetic operations and arrive at specific results.
The software needs data to be able to calculate probabilities, and a human, in turn, provides it with this data. Finally, a person will use the resulting insights.
Machine learning is, therefore, inconceivable without human intervention.
Digital tools prepare the ground for strategic decisions in sales.
Regarding the study mentioned above and the administrative sales activities, possible potentials can be identified that Machine Learning can leverage in sales.
What would your sales representative do with 60 % more time? Just imagine they employ a machine learning algorithm that predicts which customer might buy, churn or accept a higher price.
Customer behaviour has changed. It makes sense to change the way salespeople work as well. Buyers have today a relatively extensive prior knowledge of products and services and only need your sales force when they are ready to buy.
Customers gather information about vendors via the Internet and are, therefore, content-driven. This new behaviour influences how sales staff and especially decision-makers in sales should plan their sales strategies.
In other words: modern, digital tools prepare the ground for future sales decisions, which in the end still affect decision-makers, i.e. people.
Digital tools, for example, in the field of predictive analytics, will make it possible for the sales force of the future to be data-driven. Based on data, salespeople will be able to understand and anticipate their customers better. They can identify opportunities for new business, for cross- and up-selling, but also risks, such as the customer attrition.
However, data available to sales without the use of sales machine learning is limited to their own experience, intuition and possibly the strategies the company prescribes.
Whether this is always bad depends on the individual value judgements. At least in the future, the interpersonal level will continue to play a role wherever seller and buyer are or must be in direct contact.
Buyers still want to communicate directly with sellers.
Companies can, therefore, use machine learning to answer fundamental strategic questions: Which sales opportunities should you pursue? Where should we invest our time?
You can use special predictive analytics software to create differentiated customer profiles and make statements about the probable behaviour of customers. That means the sales department can provide customers with the “right” offers for them.
By combining sales and customer data, it is possible to determine the characteristics and behaviour of buyers that are statistically related to a specific sales performance.
Studies on the B2B sector have shown that the majority of potential buyers still like to communicate directly with a salesperson when researching new products and services. Communication from person to person, therefore, continues to play an important role. The situation is different when rebuying the same product. Here, there are far fewer buyers who seek direct contact with the seller.
CALCULATE NOW THE ROI OF QYMATIX PREDICTIVE SALES SOFTWARE
Use data analysis methods with a cool head.
Essential for the classification of procedures such as predictive analytics or machine learning in general is: They have the undeniable advantage of relieving sales teams of repetitive, administrative tasks. They can analyze more data than a human being could ever do. And they make it possible for companies to understand the preferences of their users and make recommendations based on this data.
However, one should be careful when saying that data can never be wrong. Algorithms and AI can also be wrong. Just like all the sales knowledge that a salesperson acquires, the software cannot guarantee future sales success. With this in mind, companies should also use appropriate procedures.
As support for sales, as a possibility to gain new insights from existing customer data, which might not have been possible otherwise. But not as a miracle cure, with which sales explode from one day to the next.
Wherever sellers and buyers meet directly, where people buy from people, statistics and formulas ultimately play a subordinate role. Every person, every buyer is unique and has different tastes and preferences. You cannot reduce these to one straightforward method.
How relevant ist the topic machine learning in b2b sales to you? Comment below!