Predictive analytics – how much data do you really need?
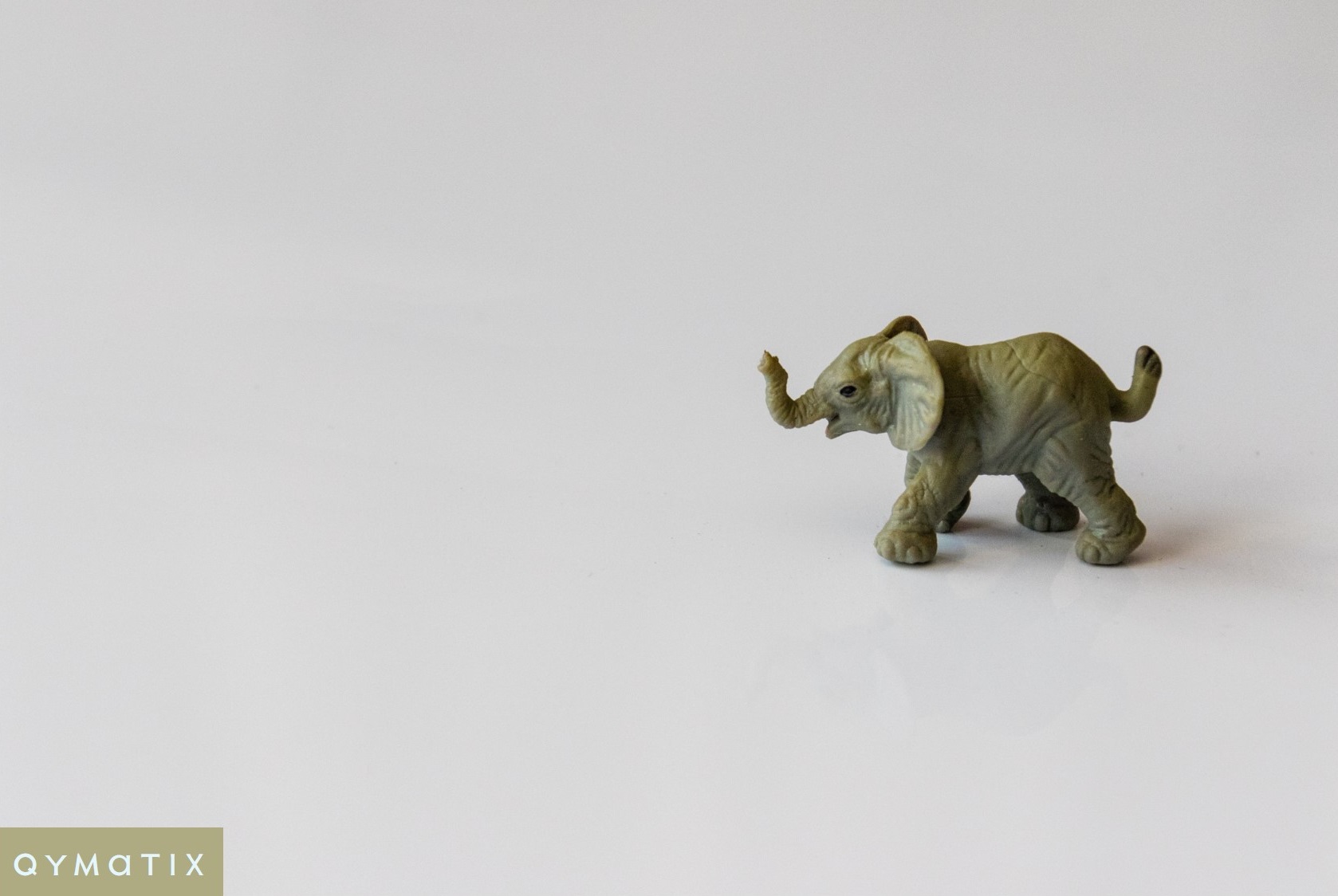
How Much Data Do You Need For Predictive Analytics?
Predictive analytics is one of the technologies with the highest financial impact in B2B sales. Several popular applications of predictive analytics are becoming “must-have” nowadays.
Sales leaders rely on lead scoring, customer attrition modelling, cross-selling analytics and pricing analytics to prioritise their sales activities and increase their customer lifetime value.
These predictive analytics methods help sales representatives to focus on a limited number of accounts with higher chances of closing new deals, churning, paying more or up- and cross-selling.
Predictive analytics requires well-thought business cases, powerful sales analytics software and sales data. Today we write about this last point.
There is the common misconception that sales managers “do not possess enough data” (whatever that means) or that they unavoidably need to “acquire external sources of data”.
Is it so? How much data requires predictive analytics? How often do you need additional sources of data? Let’s discuss these ideas in detail.
Not black and white – Data is a medium to ends.
Your sales controlling has determined that cross-selling is a priority or that customer attrition is too high and wants to reduce it. In each of those cases, the first question that often arises is: do we have enough data to discover sales potential or reduce customer churn?
The question is, however, flawy formulated. Is not about having enough data or not. The real point is, how high is the improvement in cross-selling or attrition (or any other predictive analytics method) that you can expect, using the current sales data that you have? Do you have a solid business case?
Sales data is always mean to an end. The end is improving the financial performance of a business. How can you understand whether the data that you already have will help you to achieve this improvement?
Predictive analytics in B2B sales represents solid business cases.
What kind of sales data you already have? In Business-to-Business, most of the companies are in possession of customer records or sales transactions. These datasets usually come from CRM and ERP systems or similar. In many cases, companies store sales data across different databases, including excel and old SQL databases. A manager can use any of these datasets for predictive analytics.
Predictive analytics? It helps if you have the right goal and informative attributes.
How to know if you can use the data that you have for predictive analytics? Reasonably simple, in three steps: define the predictive analytics method you plan to use, select the sales data available, and identify the data attributes.
From the last to the first point above. What is an attribute? In data science, an attribute represents a characteristic describing a group of elements. For example, if you are using CRM data, the customer location (country, address, etc.) could be an attribute. If you are using ERP sales data, the products your customers are buying could be attributes. If you have a list of sales opportunities in excel, an attribute can be whether they are won or lost.
Depending on your selected predictive analytics method, you need to find one of the attributes on your data that you can use as a “goal attribute”. Again, if you have a list of sales plans or opportunities, and you want to close (win) more of them, intuitively the “win or lost” attribute is your goal attribute. It is the main characteristic of your data record regarding your predictive analytics application.
Predictive sales analytics requires a solid business-case and good-quality data.
Similarly, if you want to define customer churn, you will need to find an attribute called “churn” or attrition. Since you probably might have this, you can define a situation or customer status that represents your goal attribute, for example, customers not buying for a certain period. In this case, something like “customers without sales this quarter” can be your goal attribute.
Use the sales data that you already have for predictive analytics.
Additional characteristics of your sales data will – hopefully – be informative attributes. Informative attributes are those offering information about the goal attribute you selected.
For example, considering customer attrition again, the average number of transactions per quarter could be an informative attribute. Similarly, for a pricing analytics method, the average price paid cross all product portfolio might be an informative attribute.
Once you have informative attributes and an explicit goal attribute to predict, you will need data. Here we refer to the amount of data, for example, the number of ERP records, sales opportunities or CRM activities. Do you have enough data?
Sales data is always mean to an end.
The question is not only how many sales records, transactions or data points you will need, but also whether you have enough positive target elements. There is no exact number to answer this question. You will need sufficient positive cases (closed sales opportunities or churned customers) and adequate informative attributes.
What are the positive target elements? If you want to implement lead scoring based on predictive analytics, positive target elements will be “won” leads. Similarly, if you’re going to reduce customer churn, positive elements will be customers that have already churned. Based on these target elements, a predictive analytics software will calculate the information it is present on your features.
CALCULATE NOW THE ROI OF QYMATIX PREDICTIVE SALES SOFTWARE
How much data do you need to implement predictive analytics? – Summary.
To the question: “how much data do we need for predictive analytics” there is a simple answer: “not much”. Furthermore, the amount of data depends on the predictive analytics method, the selected business goal and data quality.
Unfortunately, many companies still falsely believe that they “do not possess enough” data for predictive analytics. It really depends.
Predictive analytics in B2B sales represents solid business cases. Companies should define this business case and the select data “the closest” possible to their goal. For example, if the reduction of customer attrition is the business goal, sales data from an ERP will be the “closest” possible data, followed by sales orders and sales leads.
Once a company has determined what kind of data they need for predictive analytics, they can evaluate whether they might need external sources of data. They should base this calculation on a cost-benefit analysis.
We have been working in Predictive Analytics using artificial intelligence for the past years and so far, never encountered a B2B sales team without enough data. Write us a message if you want to discuss with us the possible application of predictive analytics into your sales organisation. We are happy to help.
CONTACT US TODAY FOR A LIVE DEMO
Free eBook for download: How To Get Started With Predictive Sales Analytics – Methods, data and practical ideas
Predictive analytics is the technology that enables a look into the future. What data do you need? How do you get started with predictive analytics? What methods can you use?
Download the free eBook now.
- We will use this data only to contact you for discussing predictive sales KPIs. You can read here our declaration on data protection.
