Four Machine Learning Applications Your B2B Sales Teams Might Be Using Today
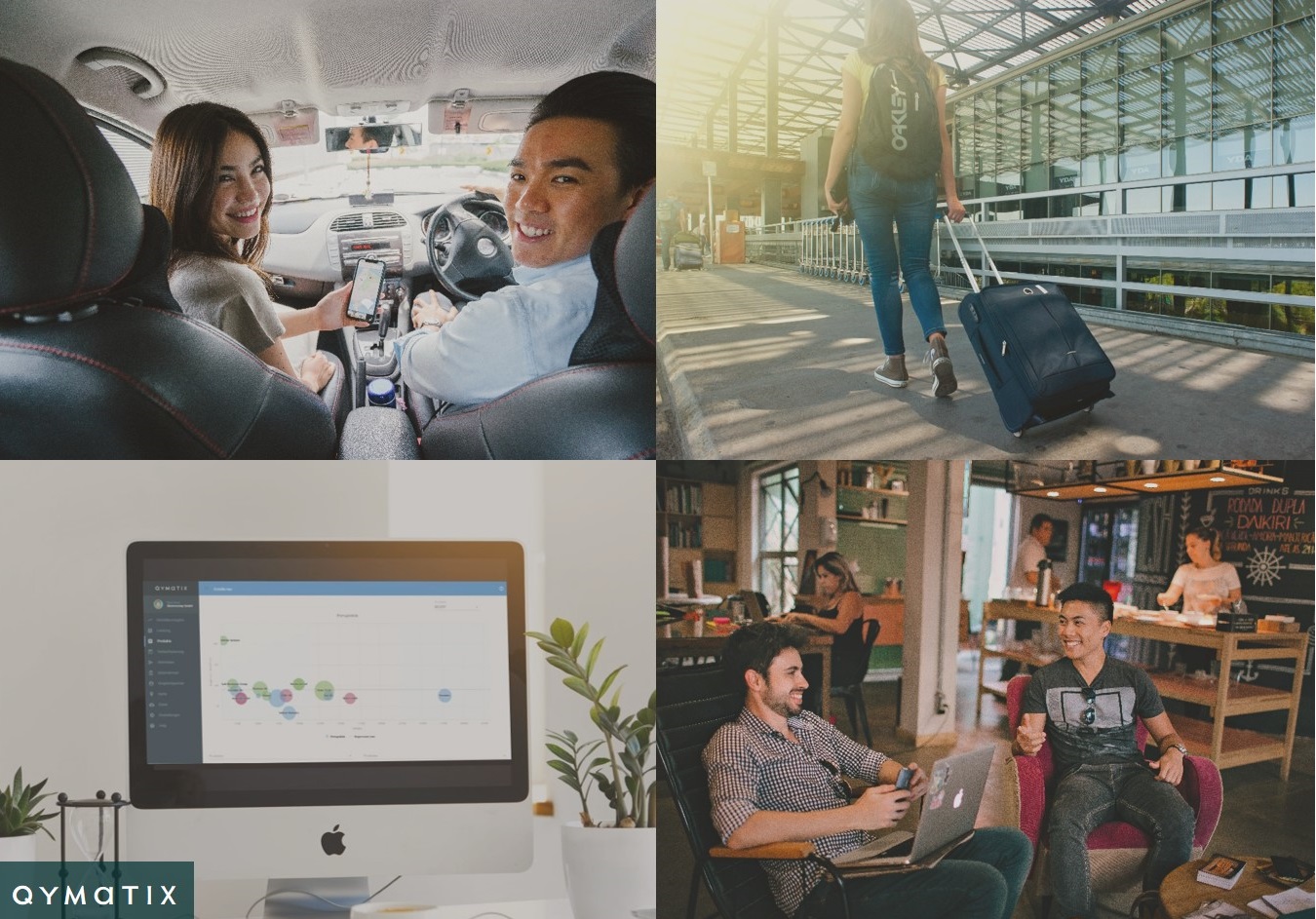
Real life machine learning examples for B2B sales
Without any doubt, in the coming years, progress in machine learning, artificial intelligence and sales automation, will replace many of the time-consuming tasks of B2B salespeople. Your sales team is probably already using machine learning.
Artificial Intelligence (AI) in sales does not mean that Terminator is applying for a sales job, no. It mainly means machine learning. Machine Learning (ML) is a part of what researchers call “narrow” or “weak” artificial intelligence. Neither “weak” or “narrow” sound much of a fighting robot. Weak AI uses software to solve specific problems better than humans.
If your sales team work on B2B, they are most likely already using one or more software using machine learning. Soon, many more will be ubiquitous. Why? Because machine learning plays a central role in making sales teams more efficient.
With ML software salespeople can find their way to a customer faster, buy cheaper flight tickets, translate a technical manual, prioritise incoming inquiries or accounts, and predict which customers might stop buying.
Are you familiar with these machine learning applications? Maybe more than you think. Let’s review them in more detail.
Do your Key Account Managers travel to customers? They are most likely using machine learning.
Intelligent driving assistant systems use machine learning to optimise routes and travel times. Most smartphone owners use navigation apps, and most prefer Google Maps, according to a recent survey. This machine learning powered application has two-thirds of the American market.
To feed its machine learning algorithms, Google Maps relies primarily on the users’ smartphones. Salespeople (as most users) provide Google Maps with information about their location, itinerary and travelling speed.
All the information the users gives to Google Maps helps them to estimate traffic and possible congestions. Furthermore, it helps the software to make accurate predictions based on historical data. Google is developing some state-of-the-art machine learning models that other companies can apply, for example random forests.
Google is developing some state-of-the-art machine learning models that other companies can apply, for example random forests.
After the acquisition of the navigation start-up Waze in 2013, Google Map started to diversify its data sources. It now allows users to report accidents, bottlenecks, and traffic as they drive. It also partners with local city authorities to produce accident data, construction updates, and road closures.
Quo Vadis, sales?
Is your team working on international sales? They are using machine learning applications for flights pricing.
If instead of driving, your Key Account Managers need to flight to their customers, then they (or the sites where they buy plane tickets) are using machine learning for pricing. Please do not think that robots are trying to rip you off, no. Airlines use this technology to increase the value they can extract from their assets.
Carriers use complex policies to set prices. The airline industry has become one of the most sophisticated users of dynamic pricing strategies with machine learning, to maximise revenue in a very competitive market.
Studies and applications of machine learning in the airline industry have been around since before the dot-com bubble. One well-known research in the area is “To Buy or Not to Buy: Mining Airfare Data to Minimize Ticket Purchase Price”. This paper observed in new detail the evolution of prices according to flight number and itinerary.
A more recent study compared two machine learning methods for pricing analytics using several attributes: seat classes, sales channels, seasonality, availability of seats, among others.
A random forest (a learning method for classification constructing a multitude of decision trees) correctly predicted price developments in 95.2 % of the cases – compared with 69.0 % of linear regression.
Does your sales team need to use several languages? The chances are that they are using machine learning for their translations.
This very blog is partially being translated using machine learning software (who would have guessed, right?). Odds are, that if your company is working in a field of sales that requires talking to customers in other languages than your local one, it might be using machine learning as well.
Yes, machine learning is also in use for translations. Computers can quickly learn words and patterns of both original and target language. They become better with the time although they cannot still understand and translate many of the idiomatic expressions and grammar exceptions.
Two recently published research papers detailed the use of unsupervised machine-learning methods for translations. These two independent studies used similar methods. Both built dictionaries without human intervention, using words with the same meaning across different languages, such as “shoe” or “rock”. This trick enabled the AI solution to find clusters and relationships between words.
Parlez-vous artificial intelligence?
Predictive Sales Software Using A Random Forest To Forecast Which Customer Is About To Churn.
In the quest to sell more, many businesses forget about not losing customers or react, once a customer has stopped buying. It is significantly more successful in defining customer retention strategies based on predictive analytics.
Using a combination of multilinear regressions and a random forest, Qymatix Churn Software can group customers by the probability of not buying again – defined as churn or attrition risk. The algorithm works correctly in 70 to 90 % of the cases and can help to cut customer churn in half.
The algorithm works correctly in 70 to 90 % of the cases and can help to cut customer churn in half.
B2B companies use this machine learning application for customer attrition (or churn risk) to find and prevent hard and soft attrition across hundreds of customers and products.
We apply the same predictive analytic method (with proprietary alterations) to pricing analytics and lead scoring. We use comparable non-supervised machine learning methods for cross-selling analytics.
CALCULATE NOW THE ROI OF QYMATIX PREDICTIVE SALES SOFTWARE
Four machine learning applications your B2B sales teams might be using today – Summary.
Do not get befuddled by claims of artificial intelligence. AI researchers classify machine learning as a weak AI application. There are clear real-world examples of machine learning that your salespeople might be using today.
Whether they travel to customers by car or by plane, they are probably using machine learning. These algorithms support a more effective decision-making process.
Machine learning generates knowledge based on data. In the case of text-translations, it uses dictionaries and past translations. In the case of sales analytics, it uses recent sales transactions.
Is there any other application of machine learning that you are using today in B2B sales? Please comment about it here.
CONTACT US TODAY FOR YOUR PERSONAL CALL
Free eBook for download: How To Get Started With Predictive Sales Analytics – Methods, data and practical ideas
Predictive analytics is the technology that enables a look into the future. What data do you need? How do you get started with predictive analytics? What methods can you use?
Download the free eBook now.
- We will use this data only to contact you for discussing predictive sales KPIs. You can read here our declaration on data protection.

More about B2B Sales & Machine Learning:
Sales Management in times of Artificial Intelligence – Five tips to redefine B2B Sales
Four Machine Learning Applications Your B2B Sales Teams Might Be Using Today
Further Read:
To Buy or not to Buy: Mining Airfare Data to Minimize Purchase Price