Why AI-based B2B Sales Forecasting is Important and Still Fails
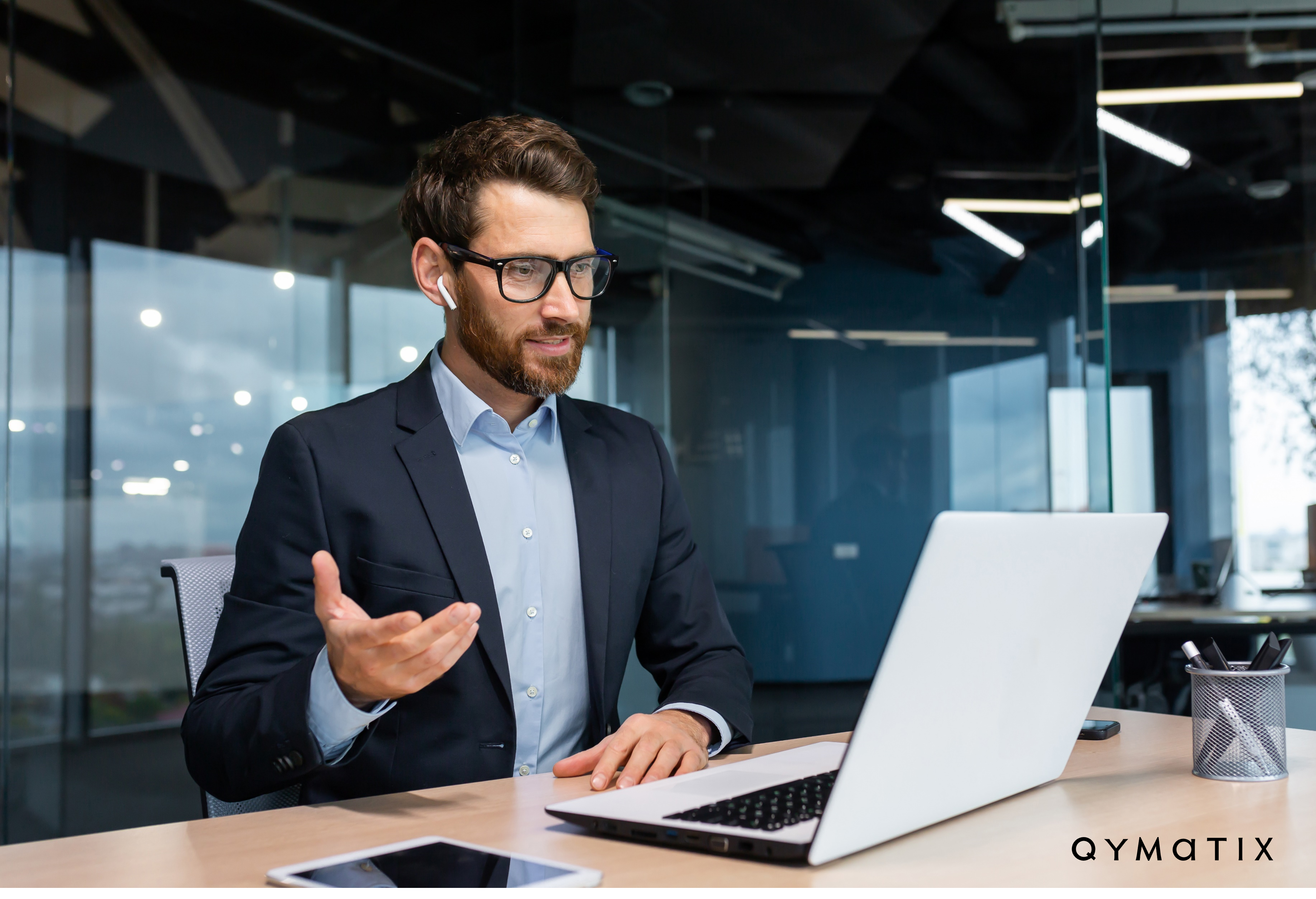
Please enter your Email address
Do you want to realize your potential in B2B sales and be more successful than the competition? Accurate AI-based b2b sales forecasting is an insider’s tip – right?
To help you understand what you should know primarily about b2b sales forecasting, I will quote Editor-in-Chief Michael Gilliland, who has published several articles in “The International Journal of Applied Forecasting.”
“Our forecasts are never as accurate as we would like them to be or as they should be. The result is a great urge to throw money at the problem in the hope that the problem will go away. There are many consultants and software vendors out there who will scoop up the money in exchange for promises to improve forecast quality, but those promises still need to be fulfilled. Many organizations, perhaps including yours, have spent thousands or even millions of dollars on the forecasting problem, only to end up back with the same lousy forecast.”
Sounds very motivating. The quote does not mean to be an introduction to an anti-forecast article, but it reflects the reality in many companies.
Some company exceptions have already successfully incorporated sales forecasting into their sales process. But these companies are the pioneers and, so far – exceptions. So, what is going wrong?
Let us get right to the one thing you should know about forecasting: Forecasts don’t get better just because they are done with ever-increasing (and expensive) effort. Never trust a 100 % accurate prediction. Even the most elaborate forecast cannot not achieve 100% accuracy. That is the nature of forecasts because no human or machine has supernatural powers to see into the future. Forecasts are calculations of probability. And they are effective when used correctly. We do not have a quality problem so much as an implementation problem.
This article discusses why sales forecasting fails and what you can do to become a successful leader using them.
Why are Sales Forecasts Important?
If you’ve already seen the benefit of accurate sales forecasting, feel free to skip a paragraph. But understanding the potential of forecasting in sales is the basis for this article. Why are so many companies trying to incorporate a forecasting process into sales?
Accurate sales forecasts hold enormous potential. The most successful and well-known example is Amazon: the giant’s cross-selling algorithm accounts for 25% of sales. “You might also be interested in…” or “Other customers also bought…” are just one example of a probability calculation for likely future purchases by Amazon customers. Haven’t you clicked on such a suggestion before?
Especially in the B2B wholesale and distribution sector, sales forecasts are enormously valuable and create a competitive advantage you should be aware of. Over thousands of customers and products, predictive sales software determines the most promising actions for sales, from optimal pricing to churn risks and additional sales opportunities. A tremendous monetary potential is hidden in your data and uncovered by machine learning algorithms.
Here I list the main benefits arising from sales predictions, briefly enumerated as follows:
– Finding the products with the highest purchase probability per customer, increasing sales and customer satisfaction.
– Prioritising customers with the highest overall purchase potential.
– Predicting the optimal final price for a specific customer and product combination.
– Focusing your valuable sales resources where they have a higher leverage.
– Data-based recommendations provide planning reliability.
– Gaining a competitive advantage – is your competition already using sales forecasting for sales planning? Then it is time to catch up.
As you can see, you should be aware of sales forecasts, and it is only logical that many companies invest in this. But what leads to their failure?
1. Sales Forecasts as a “Never-Ending Story”.
As indicated in the introduction, more resources do not lead to significantly better predictions. Understandably, in times of Big Data, executives dream of using all information to improve forecasts: “Let’s add social media data!” or “What about the current market data from China – we need that too!”.
Successfully using sales predictions starts with the question, “what data do we need?” before you build a forecast model.
And here’s our tip: first and foremost, sales forecasts need historical data close to the target. In other words, historical sales data. B2B Distributors and wholesalers have this data in their ERP systems, and it is worth its weight in gold. Because none of your competitors has your sales data (hopefully), it is reliable, free to use and unique.
You should remember that when it comes to data for forecasting, you should always weigh the costs and benefits. External data, such as market or social media data, is hard to get, must be maintained and updated. And what benefit do they bring? Mining your ERP sales data alone can already generate sales forecasts with up to 95% accuracy. A 1% improvement in accuracy, it is not worth the effort. It only costs time and resources, turning sales forecasts into endless stories.
2. Trust in Forecasts: “That’s bingo!”
Confidence in sales forecasts is bigger than this article – scope for a scientific paper. It’s also tricky because, at some point, companies need to put the forecast models into use.
Especially with machine learning models, forecasts get steadily better with use as the models learn through additional data. But here is the problem: especially at the beginning, users have a lot of scepticism. The sales department is constantly confronted with new technologies, such as a new CRM system, and is “a burnt child”. Doubts are, therefore, not necessarily ill-intentioned but arise from bad experiences.
That means the tolerance for “wrong forecasts” is not very high. Do you remember that no prognosis model in the world gives exclusively correct recommendations? So even a best-trained model will provide forecasts that turn out to be “wrong” in hindsight. In addition, in our hypothetical scenario, we are just at the beginning, and the forecast model has yet to reach its full potential.
After two or three times of half-hearted trial and error, sales reps and associates start smiling at the forecast recommendations, and the whole thing is down the drain. Lost. The forecasts have failed. Even “correct” predictions cannot save this anymore because the employees label them as coincidence: “That’s bingo!”.
Too bad, because it’s not.
What to do? We conducted a few interviews with companies that have successfully employed a sales forecasting software. First, the sales leader or manager had to gain confidence. Some reported that they put the software through its paces by comparing its results to their own Excel spreadsheets. That allowed them to better understand the results of the software in the first place, and in the next step, they realized that the software also recognized the potential that the Excel spreadsheet did not show.
The next step concerns the sales team. Start with a small group of your employees who are open-minded about modern technology – a selected test group. Remember that a predictive sales model or software generates thousands of recommendations. That is overwhelming. Give this test group a small number of daily recommendations with a high probability of success (e.g., five). After some time, compare the results of the test group with the rest of the employees. This way, you can see financial results with and without a sales forecast. Numbers create trust.
3. Sales Forecasts Must be Integrated into the Sales Process.
Let’s move on to the next implementation problem: “Hello, dear customer; my algorithm says you won’t want to buy from us soon. Is that right?” — an interesting start to a customer engagement conversation. You can guess what the next sales prediction problem boils down to. “Good to know, but now what?”.
Sales forecasting tells your sales team, “Look out; customer X is highly likely to churn.” Or “Look, customer Y might still be interested in this product.”.
Now, if every time you make a sales forecast, every one of your sales reps must start thinking about how to get that to the customer, it takes too much time.
There needs to be a process. A clear path for what a sales rep needs to do and when. The first thing to decide is which prediction needs action and which one not? The picture shows an exemplary process, which you can, of course, adapt to your company.
If you decide to implement an action, you can define further steps: Was there a cross-selling recommendation? An e-mail with an offer may be enough. Is a customer at risk of churn? Start your customer loyalty measures. It is also always advisable to define a good conversation starter, such as if the customer is due for a maintenance appointment soon.
As you can see, there are many possibilities, and they are as individual as your company. But integrating such forecast processes into your daily sales routine is worth it.
CALCULATE NOW THE ROI OF QYMATIX PREDICTIVE SALES SOFTWARE
Why AI-based Sales Forecasting is Important in B2B and Still Fails – Conclusion.
Briefly, forecast processes in sales do not need to fail. Properly implemented, sales forecasting holds enormous potential and is essential for the future of B2B sales. Both the B2C sector and companies in the USA are already demonstrating this today.
Predictive sales analytics is on the rise in Germany right now. Do you remember how many failed CRM projects there were – or still are? Nevertheless, a CRM system is indispensable for companies above a specific size. So, it will be for AI-based sales forecasting.
I WANT PREDICTIVE ANALYTICS FOR B2B SALES.
Further Read: