Understanding Artificial Intelligence: Real vs. Fake AI
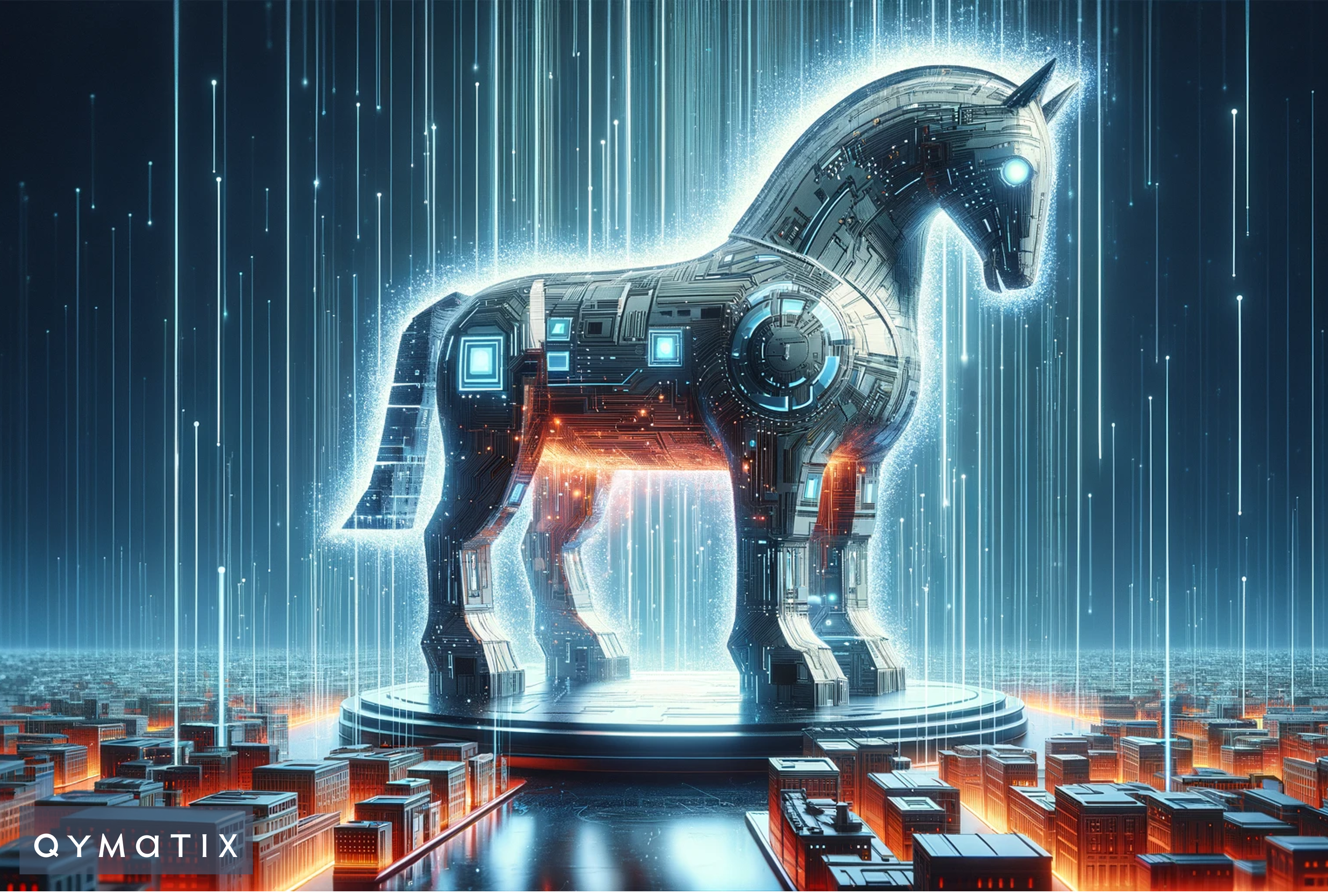
Please enter your Email address
Is there real and fake artificial intelligence?
Artificial intelligence (AI) has attracted enormous interest and attention in recent years. From autonomous vehicles and speech recognition to personalized recommendation systems, the potential applications seem endless. But amidst the excitement of advances in AI technology, one question is increasingly being asked: Is there such a thing as fake AI?
The term “fake AI” refers to applications that appear intelligent at first glance but lack “real” artificial intelligence.
These applications often use simple rules or pre-programmed models to perform specific tasks. They lack the machine learning and adaptation capabilities considered core “real” AI elements.
Is there a Definition for “Real AI”?
Distinguishing between “real” and “fake” AI is complex because there is no universally accepted definition of artificial intelligence. We already fail to define “intelligence”. There are many attempts at definitions by various experts.
Elaine Rich, for example: “Artificial intelligence is the study of how to make computers do things that humans are currently better at doing.”
The beauty of this definition is that computers are associated with specific tasks. These tasks include image recognition, speech understanding, text recognition, and prediction based on experience.
As a result, there is much debate about “fake AI” and the definition of real AI.
Some argue that true AI must be able to learn independently and continuously adapt to new data and environments. Others emphasize that the definition of AI should be based less on functionality and more on the ability to make intelligent decisions.
Despite the lack of a precise definition of artificial intelligence, there are some examples that are not regarded as AI (i.e. as “fake AI”) by the majority of AI experts:
1. The Illusion of Intelligence:
A prominent example of “fake AI” can be found in some virtual assistants and chatbots. They can appear sophisticated and even understand natural language, but their “intelligence” is often based on predefined scripts. They are limited in understanding and cannot learn independently or solve complex problems.
2. Rule-based Systems:
Traditional rule-based systems are also often considered “fake AI”. These systems work according to predefined rules and instructions without the ability to adapt or learn on their own. They depend on clear instructions and cannot react to unforeseen situations.
3. AI Washing:
Another aspect that contributes to the confusion is so-called “AI-washing”. That is the conscious or unconscious overemphasis of AI elements in products or services to make them appear more modern or advanced. For example, companies may sell simple automation processes as AI integration when the underlying technology is limited.
The Risks of “Fake AI”
The existence of “fake AI” poses certain risks. Exaggerated expectations can arise if companies or users believe that an application has real AI capabilities when it does not. That can lead to disappointment and loss of trust. For this reason, transparent communication about the functionality of different technologies is essential. It is crucial to know what a technology can do and where its limitations lie.
Is only Generative AI “Real AI”?
Since generative AI capabilities have been made available to the general public through chat GPT, there has also been discussion about whether only this type of AI should be considered “real.”
Generative AI refers to models that can create new data or content, whether in the form of text, images, or other media. These models, particularly those based on neural networks, can demonstrate creative capabilities by learning from patterns in the data and generating new, previously unseen content.
It is important to note that generative AI is only one facet of the broad spectrum of AI technologies. There are also other categories, such as reactive AI, which is based on fixed rules and performs pre-programmed tasks, or self-learning AI, which continuously learns from experience and data.
The definition of “true AI” should not be based solely on the ability to generate new content. Real AI could rather be seen as a system capable of acting intelligently, learning, understanding, and solving complex problems. This includes adapting to new situations, extracting knowledge from unstructured data, making predictions, and continuously improving.
Generative AI is undoubtedly an advanced form of AI, but it would be too restrictive to exclude all other categories. A holistic view should consider the diversity of AI approaches to capture the broad range of applications and possibilities in this rapidly evolving field.
Ultimately, the question of what is considered “true AI” lies in the definition and expectations of AI systems.
What Companies should Consider
Much more important than whether it is “real AI” is the question of the company’s own application goals and expectations. Many companies don’t want to miss out on the new AI trend and say, “We have to do something with AI!”
However, as we know from Elaine Rich, artificial intelligence systems are also tools for specific application problems.
That means it is important to understand what goals the system should achieve in your company. In some cases, simple automation mechanisms or rule-based systems may be completely sufficient. In other cases, when it comes to complex problem solving, very large data sets, or autonomous learning, artificial intelligence may be required.
Also, think about adaptability to future requirements. If the requirements for a system are likely to increase or change over time, you are well advised to use artificial intelligence. Its ability to continuously adapt gives it an advantage over static systems.
CALCULATE NOW THE ROI OF QYMATIX PREDICTIVE SALES SOFTWARE
Understanding Artificial Intelligence: Real vs Fake AI – Conclusion:
It is crucial for companies to assess the capabilities of AI systems they are deploying or considering deploying realistically. This includes understanding whether these systems are actually capable of learning and adapting from data, which would make them “true AI.”
Investments in AI should be based on a clear understanding of your business strategy and specific requirements. It is important to look beyond the hype and soberly assess the technological capabilities and limitations.
Sometimes, a well-designed system tailored to specific requirements can be very effective even without “real AI”. In other cases, AI systems have clear advantages over their static counterparts. It is, therefore, important to carefully assess the specific requirements and objectives and select the technology accordingly.