What is the fuzz about Predictive Analytics? One example of B2B Sales will make you start today.
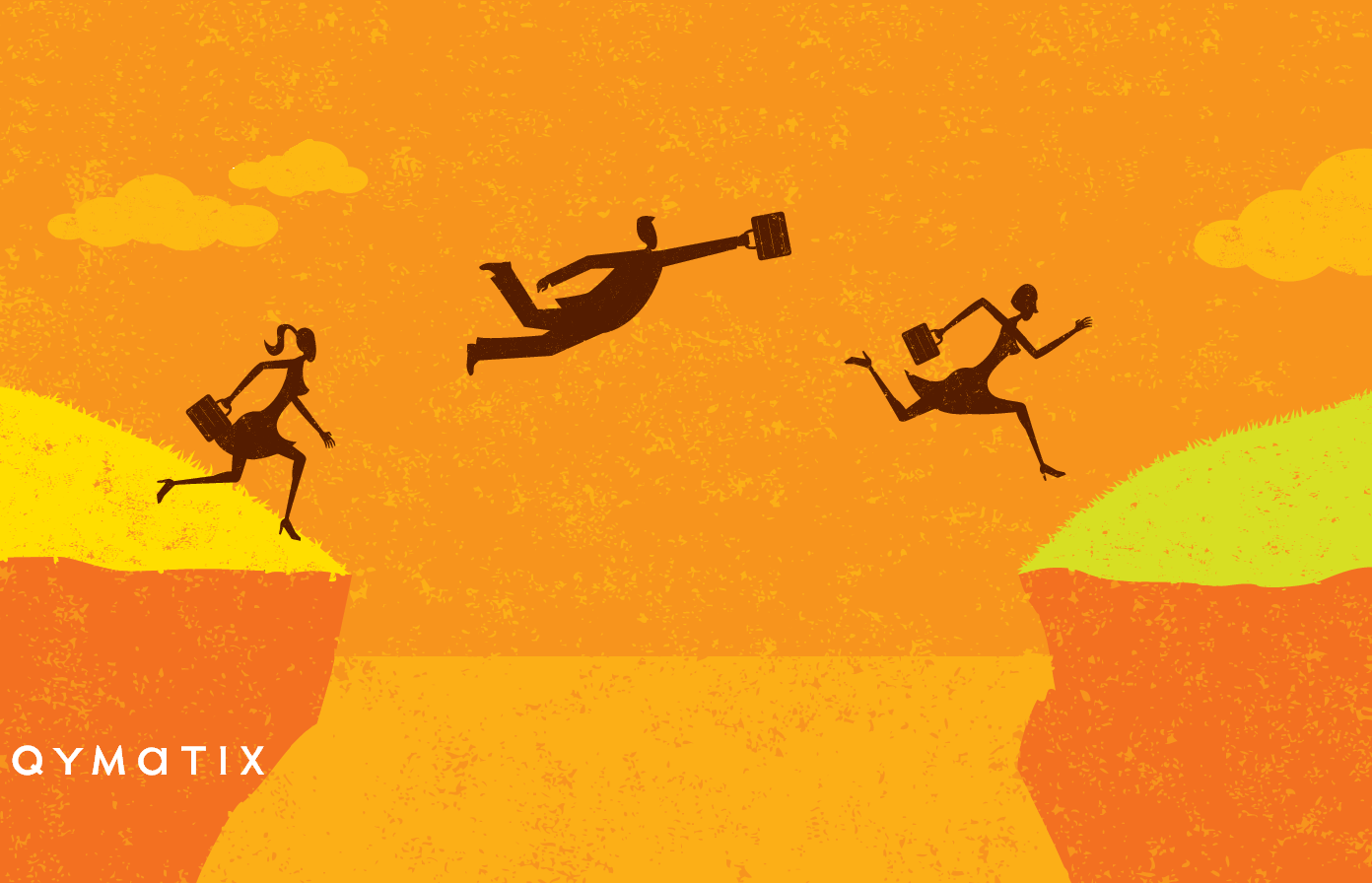
B2B sales went through several transformations in the past decade. Mobile is ubiquitous, CRM Systems are universal and “customer journey” achieved mainstream status. However, Predictive analytics is cutting a “before and after” in B2B sales.
The ability to make predictions radically changes sales in business-to-business (B2B). It brings enormous advantages to pricing, reduces churn and improves sales planning.
Predictive Analytics for Sales brings a specific monetisation to Big Data. In turn, this financial boost gives an unassailable competitive advantage. With a straightforward example, we present you how predictive analytics will revolutionise sales.
In this case, we illustrate why predictive analytics has the power to start a revolution in B2B sales. Predictive analytics is already changing how companies find customers, leads and sales reps. Predictive sales analytics increases revenues in stagnated markets. It brings double digits ROIs when investments are drying up.
B2B Predictive Analytics has the power to add an amount of value not experienced with any of the past trends. Successful predictive analytics in B2B depends nonetheless on a clear ERP data mining process. And it will only add value if controllers and sales managers consider carefully what their business goals are and how and how they can improve their sales planning process.
Lastly, executives should adapt their sales KPI to the new world of predictive analytics.
In this article, we use a cost-benefit matrix to present the monetary advantages of Predictive Analytics tools in comparison to “gut feelings” analysis/decision making.
What is the goal of Predictive Analytics in B2B Sales?
Any data mining model should start with clear goals. Unfortunately, quite often sales managers start investing in analytical capabilities, disregarding what their objectives are. Therefore, for this example, we will first define the goal of our analytical model.
Our goal is to maximise the output of our Key Account Manager (KAM) or sales rep during a month. Let’s assume, for example, that our KAM serves 100 accounts in her sales region. She would like to visit those customers who are most likely to place an order.
Let’s also consider possible datasets available for this model. First, past sales transactions, for example, taken from the company’s ERP system. We also have recent sales activities, from a CRM. Also, we might possess information regarding the target accounts: size (in employees and annual revenues), location and industry. At least one of these three datasets are available in almost any enterprise.
Example of a Cost-Benefit Matrix for a B2B Predictive Analytics Model
Now, we build a Cost-Benefit Matrix of our predictive analytics model. A Cost-Benefit Matrix represents the sum of all benefits minus the costs of each predicted situation.
For simplicity, this model has two possible outcomes only: positive (the customer buys) or negative (the customer does not buy). These results are on the horizontal axis of the matrix – the actual results. Similarly, the model predicts two possible outcomes: “yes, the customer will buy”, and “no, the customer will not buy” – if visited. The “Y” and “N” on the vertical axis represents this classification.
There are four possible outcomes:
True Positive: the model predicted that the customer would buy and indeed it bought.
True Negative: the model predictive that the customer would not buy and indeed it did not.
These are the “good” or the “right” predictions of the model.
False Positive: the model predictive that the customer would buy, but it did not.
False Negative: the model predictive that the customer would not buy, but it would have if visited!
These are “bad”, “wrong” or “false” classifications. In both cases, the model incorrectly predicted the actual or real outcome.
Usually, both “misses” or “mistakes” do not cost the same. The monetary difference between both situations is the main point of our example.
What is the cost of visiting a customer that would not buy anyway? What is the cost (or missed benefit) of a non-visited customer who would have purchased?
Let’s have a more in-depth look at that.
Predictive Analytics for B2B sales: Expected Benefits – Example
We need to define now the cost and benefits associated with each decision par. let’s give our analysis some real-world numbers with the following assumptions (on average):
Visiting each customer costs the company € 500. The KAM can visit up to five accounts a day. | |
Each deal represents € 10,000, with a margin of 50%. That is, one buying customer brings a €5,000 benefit. | |
Only 10 customers would close a deal if visited this month. The KAM doesn’t know who they are. She has a good guess but cannot know for certain. Sounds familiar? |
Although these are our assumptions, they reflect a real B2B sales cost estimation. Sales leaders can, of course, repeat the exercise with their own sales costs and benefits.
Let’s compare these two models: randomly visiting every possible customer, vs visiting those with the highest probability to buy, using predictive analytics.
First Model – Randomly visiting every possible customer based on a hunch
Nobody will be surprised to find out that this is still the most common Key Account Management method. Sales leaders and KAM usually let their experience and hunches dictate what to do next. Some of them may be successful, but surely everyone has room for improvement. Why? Let’s calculate the costs and benefits of this model.
The KAM or rep should visit 100 accounts in a month. At five per day, in 20 days, she will struggle to properly prepare each appointment. Scheduling appointments from the car and answering emails late at night – no office time.
Visiting all possible customers, and using our assumption, the KAM will close every available deal. We assumed there were 10 of them, with € 5,000 in benefits each. That means, at the end of the month, the company made a profit of € 50,000. However, during this rushing month, the KAM costs his company €50,000 (€ 500 x 100 visits).
Irrational. It cost € 50,000 to make € 50,000. This model brings zero, zilch, nada. Accounting differences aside (for example, if we include the cost of selling in the 50 % margin), this is an extremely inefficient sales method. Stressful and uneconomical.
Still, many B2B organisations push their sales reps to use it. Sales leaders can estimate their inefficiencies by reviewing the assumptions made above using real company’s figures.
One might argue that no seasoned KAM will visit all 100 customers. She will surely concentrate her efforts in a reduced number of accounts, those with higher chances of closing a deal. Well, that is – precisely – what predictions are all about, isn’t it?
The KAM will follow her instinct and experience. A predictive analytics algorithm crunches ERP and CRM sales data. Another case of “man vs machine”.
Some sales reps will also argue that they enjoy visiting their customers. That is, of course, a valid point. However, here we are not discussing what is more enjoyable but what is more productive.
Second Model: Sales planning process using predictive analytics
Enters predictive analytics. The company uses a software tool predicting the 20 accounts closing a deal this month. The KAM visits them, incurring in € 10,000 costs (20 x € 500). She can deal with one customer a day, properly preparing each negotiation and follow up.
To calculate the benefits of this second model, we need to know how many of the ten positive cases the model correctly predicted.
They are the “true positives”: accounts predicted to buy that indeed bought. They were ten. If the model manages to find them all, the net benefit will be €40,000 (10 x €5,000 – €10,000).
In effect, using predictive analytics could offer € 40,000 in benefits, mainly in savings. Compare this to the € 0 from the “visit them all” strategy. Shall the predictive sales software find only five true positives cases, it still wins by € 15,000 (5 x € 5,000 – € 10,000).
Benefits without Predictive Analytics | Benefits with Predictive Analytics |
€ 0 | € 40,000 per Month and KAM |
These benefits are for a month only, with one Key Account Manager. Multiplying this by 12 and by the number of KAM in the sales organisation, sales leaders can estimate the total benefit of applying predictive sales analytics.
CALCULATE NOW THE ROI OF QYMATIX PREDICTIVE SALES SOFTWARE
Predictive Analytics example for B2B sales- Conclusion
Predictive analytics will have an enormous impact on B2B sales. The amount of value it can deliver in a short-time is immense. It requires well-thought business cases and useful analytical models.
We also showed some limitations. For example, since every sales situation is different, estimating the benefits of a predictive model will need adjustments.
Sales leaders should first define what their business goal is. In this example, it was to maximise KAM output during a given period. Once the purpose of the predictive model is defined, sales managers should gather sales data. Sales leaders should evaluate the expected costs and benefits, to assess the ROI of the solution.
Finally, B2B organisations should instruct its sales forces to modify their behaviour based on the predictive analytics model. Companies also need to re-think the sales KPIs they are measuring. In other words, thanks to the implementation of predictive analytics, managers must define new sales KPI and sales controlling processes.
Sales leaders can apply this same lead scoring example to other applications of predictive analytics. For instance, churn reduction, pricing analytics, and cross-selling analytics. The method is, in spirit, the same. Sales reps ought to focus on a limited number of accounts with higher chances of closing deals, churning, buying from cross-selling or paying more.
CONTACT US TODAY FOR A LIVE DEMO
Free eBook for download: How To Get Started With Predictive Sales Analytics – Methods, data and practical ideas
Predictive analytics is the technology that enables a look into the future. What data do you need? How do you get started with predictive analytics? What methods can you use?
Download the free eBook now.
- We will use this data only to contact you for discussing predictive sales KPIs. You can read here our declaration on data protection.
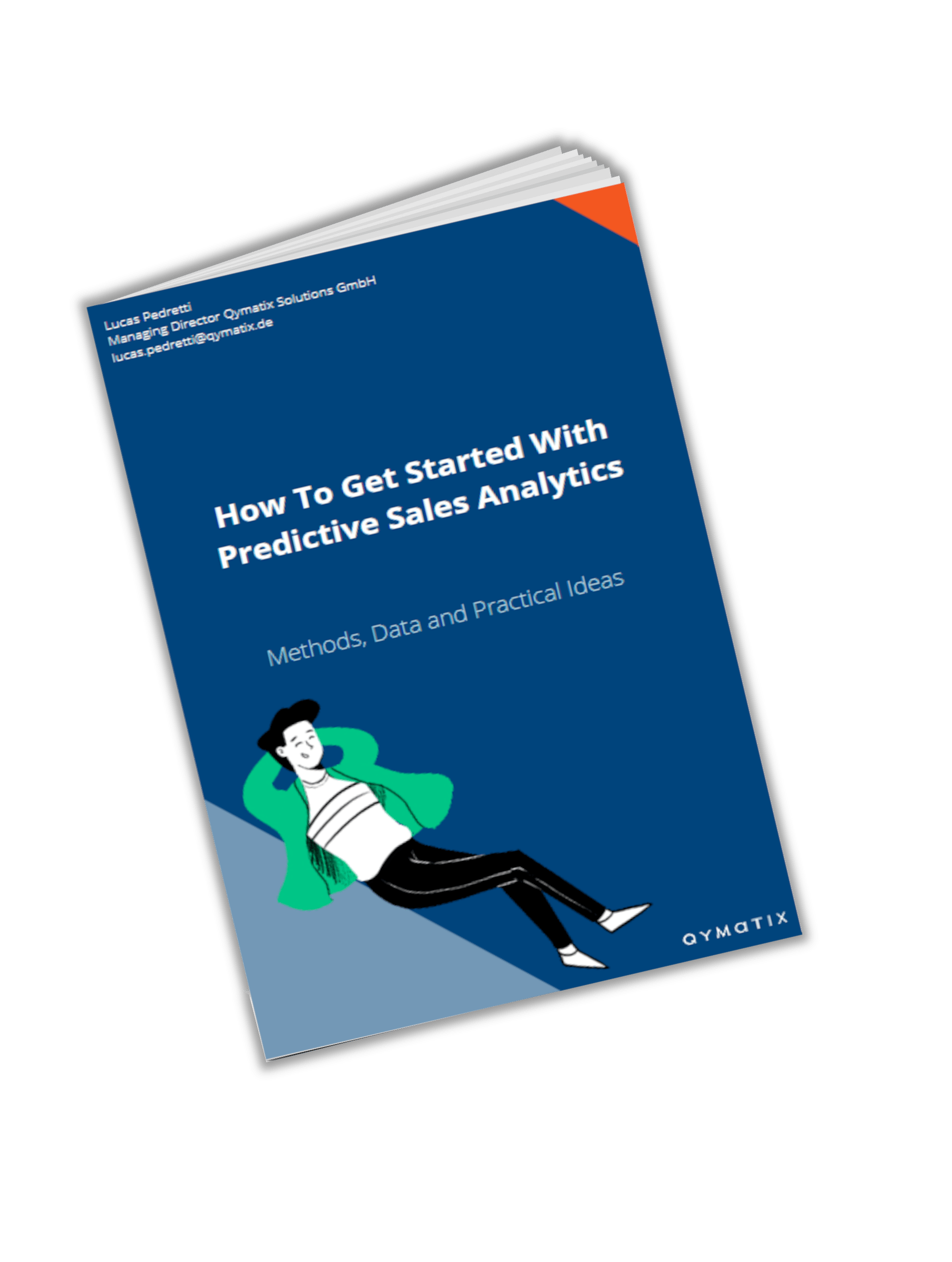
Is your sales too expensive? Read these related articles:
B2B Sales Manager Salary in Germany – How much are they doing?
Further Read:
What Big Data Mining Means for ERP Systems
Artificial intelligence for sales planning