AI Data in B2B Wholesale
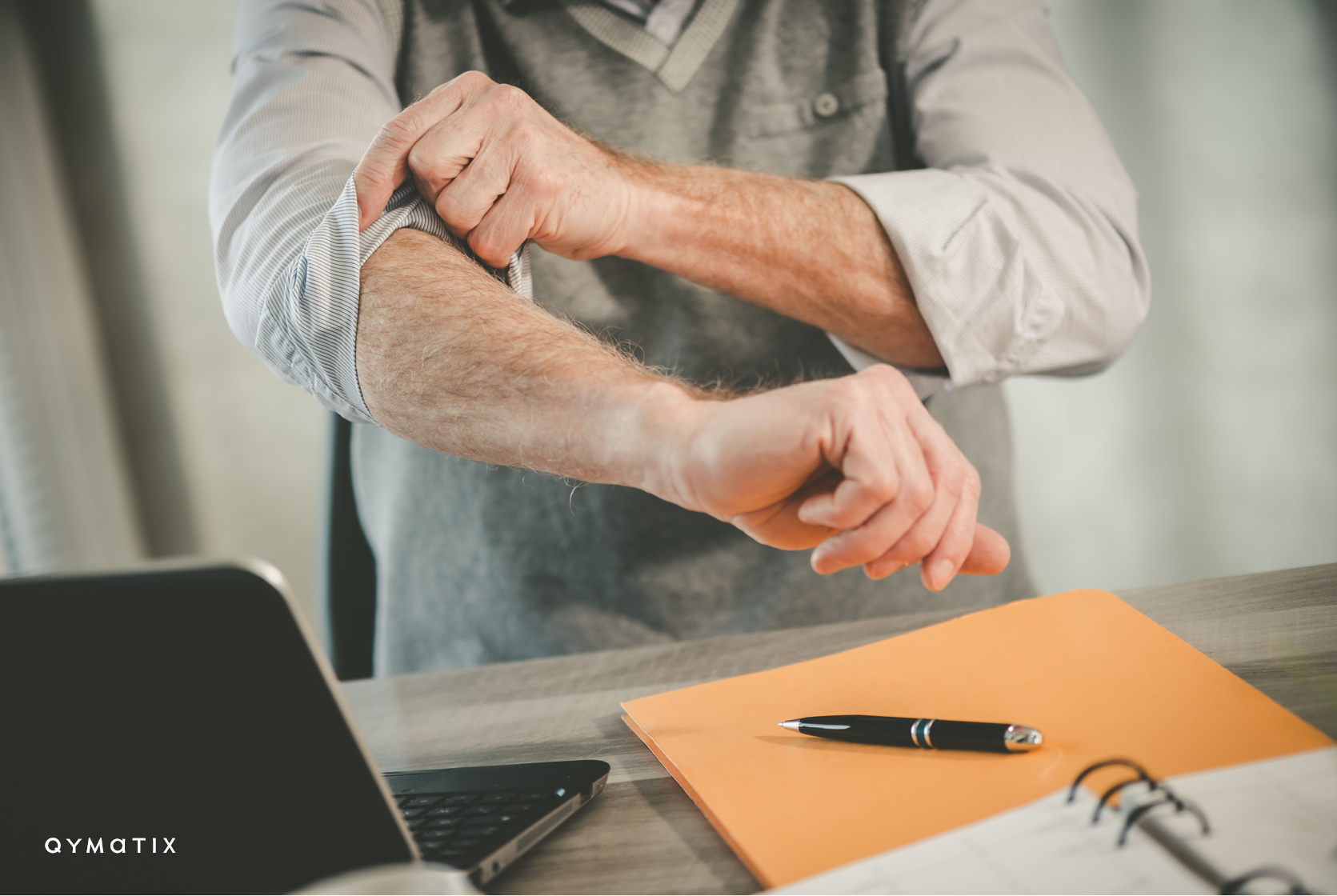
That is one of the most frequently asked questions regarding the specific use of AI applications in B2B wholesale. In this blog article, you will learn how to assess and check the quantity and quality of your data better.
You may be in a situation where you want to use your sales data to get AI-based recommendations for your sales team!
Sales Data for AI-based Sales Forecasts
Predictive sales analytics is one of the most popular AI applications in B2B wholesale. That involves making your own ERP data (sales data) usable.
A large number of customers and products characterizes wholesale companies. Together, this results in a lot of sales data. If you want to stay ahead of the competition, you cannot afford to leave this treasure trove of data unused.
AI-based predictive sales software systems (such as the Qymatix software) create sales forecasts for sales teams based on ERP data. That allows you to exploit cross-selling opportunities, initiate customer retention measures in good time if there is a high churn risk, and set prices individually.
This is based on sales data from ERP systems. What requirements must they meet to be AI-ready?
There are quantitative and qualitative requirements. We will go through the most important points step by step.
Data Quantity
By nature, most medium-sized wholesale companies will be fine with quantity. Thousands of products and customers inevitably lead to enough sales transactions per year.
Nevertheless, quantity is inevitable for AI-based machine learning. AI systems look for recurring patterns in data sets. The more data there is, the more reliably such patterns can be found.
Data Quantity Requirements
• Large amounts of data: AI models require extensive historical data to recognize meaningful patterns and make reliable predictions. For example, sales transactions must be available over several years to identify seasonal fluctuations and long-term trends.
There is no universal figure, as the exact requirement depends on the complexity of the task and the algorithms used. Nevertheless, we can give the following guide values:
• At least 30,000 to 50,000 transactions are a good starting point for an initial proof-of-concept of predictive models, such as cross-selling analyses in B2B wholesale.
• For more complex applications, such as dynamic pricing or customer churn predictions, at least one hundred thousand transactions should be available.
These numbers allow the model to analyze enough data points for different products, customers, and periods to identify reliable patterns.
• Diversity of transactions: The data set must include numerous different sales events. To train the model with a broad database, as many transactions as possible with different products, customers, and sales regions should be recorded.
• Temporal coverage: An AI model requires data collected over a long period of time. Typically, data should be available over several years to recognize both short-term and long-term developments. A rule of thumb is that at least one year’s data should be included in an initial proof-of-concept.
Overall, the more data is available in high resolution and over a long period, the better an AI model can work in B2B wholesale.
Data Quality
Data quality is a little more complicated – or let’s call it by its name: annoying.
It’s not for nothing that the saying goes: “Everyone wants clean data, but nobody wants to clean data!”
Before we get to the prerequisites, here is a tip on how to proceed if you want to start with AI in sales and are unsure about data quality:
The main prerequisite is quantity. You either have it or you don’t. Data quality is hard work.
Experienced predictive sales software providers look at your data with you and then make specific recommendations on how to proceed. In most cases, it looks like this:
• Only small “cleaning tasks”—which take half a day at most—need to be done. It is not worth postponing the AI rollout for this. Depending on the software provider’s goodwill, they may take over small cleaning tasks.
• The data requires extensive maintenance. The “clean-up work” would clearly exceed one day’s workload. In this case, hiring in-house experts or bringing in an external service provider is worth it.
Dirty or incomplete data leads to incorrect models. For machine learning, the data must be correct, complete, and free of inconsistencies. Erroneous entries (such as wrong prices or missing transaction details) falsify the analysis and predictions. That is why this “annoying” point is also important. You all know it: “Bullshit in, bullshit out.”
Six Important Criteria for Data Quality
1. Various data points (attributes) per transaction should be recorded in tabular form: details such as product name, price, quantity, customer, sales date, and region should be recorded for each sales event. These attributes should be available in a standardized table format. Good predictive sales software providers will give you a template or a direct query for your ERP system.
2. Data consistency: Data should be consistent across the entire data set. In a B2B wholesale business, for example, the same products and customers should always be recorded consistently. Inconsistencies lead to inaccurate predictions, as the model cannot interpret different terms or formats correctly.
Example: Dates in different formats (DD/MM/YYYY and MM/DD/YYYY) or product names with various spellings (e.g. “Prod-123” and “123-Prod”).
Consequences: Different formats can lead to incorrect links or misunderstandings in the model, as similar data is not merged correctly.
3. Timeliness of the data: Machine learning requires up-to-date data to keep up with the latest market developments and customer behavior. Outdated data leads to inaccurate forecasts that do not reflect these developments and market changes.
Example: Updating ERP data monthly or even weekly allows the models to reflect new trends and customer changes in a timely manner.
4. Not too much missing data (missing data)
Problem: Data records are missing individual values or entire fields, such as a sales price, customer, or date.
Detection: Missing data can be detected by analyzing the data records for null values or empty fields.
Consequences: If important data points are missing, the model cannot make precise predictions, e.g., about pricing or sales forecasts, because essential information is missing.
Example: If the price of a product is missing from a sales forecast, the model cannot correctly assess customers’ price acceptance behavior.
5. No incorrect or implausible values (outliers)
Problem: The data contains values that are outside the normal range or are obviously incorrect, such as unrealistically high or low prices or quantities.
Detection: Statistical analyses (e.g., standard deviations) can identify such values as outliers.
Consequences: Outliers can distort the model by leading to incorrect patterns that are overly weighted. Extreme values can deliver unusable results, particularly in price analyses or sales forecasts.
Example: A price of 1 euro for a product that usually costs 10,000 euros or an ordered quantity of 1,000,000 units if the average value is 100.
6. Remove duplicates
Problem: Entries in the data record are duplicated, leading to overrepresenting certain transactions.
Detection: Duplicates can be identified by checking multiple identical entries for the same fields (e.g., identical transaction numbers, customer names, or dates).
Consequences: Duplicates distort the statistical evaluations and the weighting of individual transactions in the model, which can lead to inaccurate results.
Example: A transaction is recorded twice, which falsely increases the sales figures for this product and thus generates incorrect forecasts.
CALCULATE NOW THE ROI OF QYMATIX PREDICTIVE SALES SOFTWARE
When is Sales Data in B2B Wholesale AI-ready? – Conclusion.
Most B2B wholesale companies will have no problems with data quantity, which is also decisive for an AI use case. AI-based predictive analytics systems are only profitable with a certain amount of data, as this is the only way to reveal hidden potential. Rule-based analyses using familiar spreadsheet systems such as Excel are sufficient with less data.
In terms of quality, six main criteria are important to clarify. Experienced predictive sales software providers will help you find the best way to achieve qualitative AI readiness!
Would you like to start using AI in wholesale sales but are still unsure about your data? Book an initial appointment, and we will be happy to help!