Success through Data Analytics in Sales
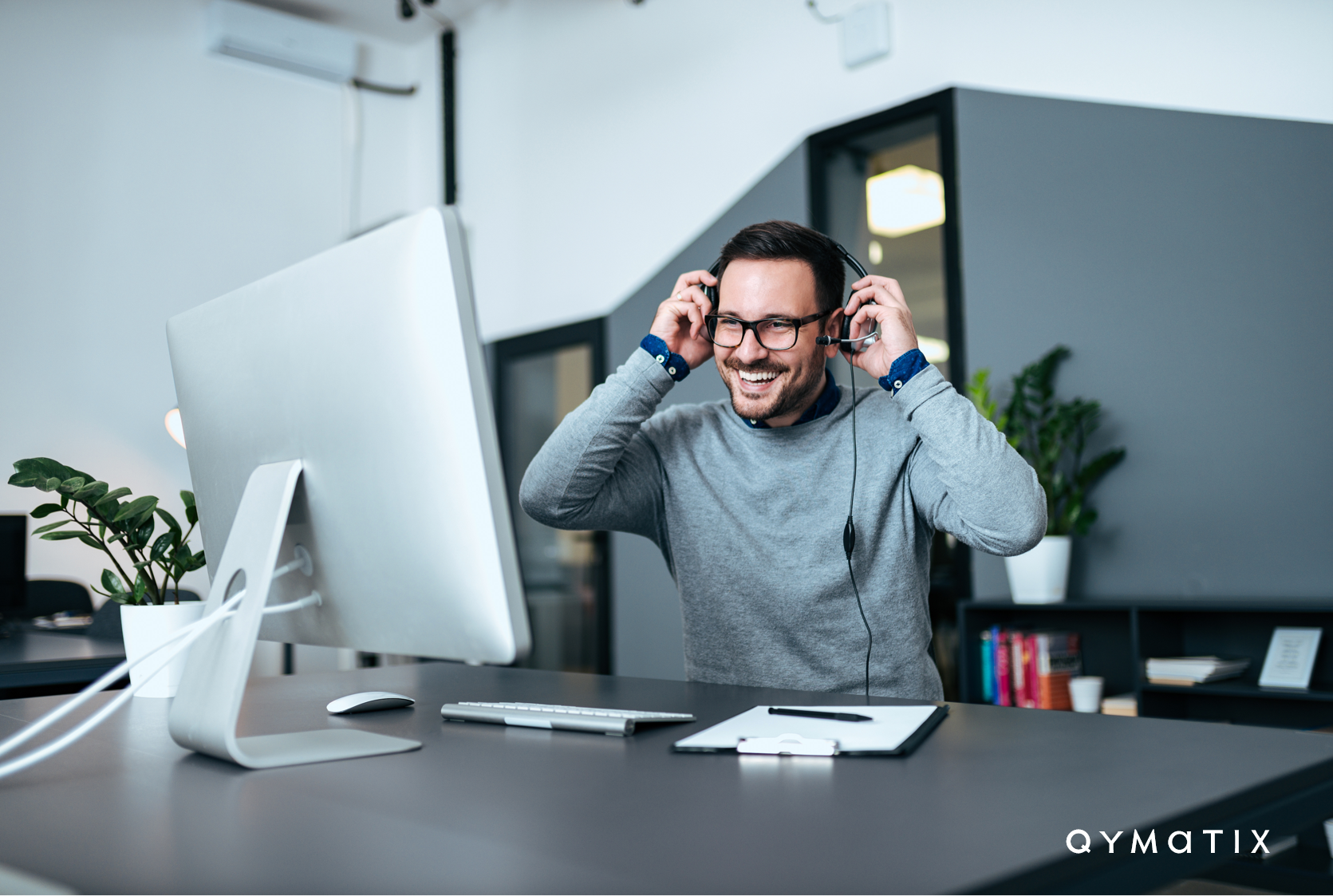
In a world where competition is tougher than ever before, data is the most valuable asset a company can have.
Targeted and effective data analysis enables not only the evaluation of current performance, but also the forecasting of future trends and the corresponding adaptation of strategies.
In this article, we discuss the opportunities offered by data analytics in B2B sales and best practices. The focus is on predictive sales analytics. We invite you to immerse yourself in the world of data with us.
The basics of data analytics in sales
Before delving deeper into the topic, it is important to familiarize yourself with the basic concepts of data analysis in sales.
The term data analysis encompasses the process of examining, cleaning and modeling data with the aim of discovering useful information, drawing conclusions and supporting decision-making.
In the sales world, this primarily means analyzing sales and customer data in order to identify patterns and develop strategies that promote sales.
Today, data analysis is an indispensable part of day-to-day business. It helps to understand current sales figures and predict future trends. When it comes to calculating the probabilities of future events, we are active in the field of predictive analytics.
A large number of successful companies have proven that targeted and effective data analysis can lead to significant increases in sales.
Types of data in sales
In order to take full advantage of data analysis, different types of data need to be considered:
– Sales data (ERP data)
Sales data includes all billing information. Aggregation provides you with information about your sales figures, your turnover, your sales volume and frequency as well as the performance of individual products or services.
Analyzing this data allows you to identify trends and patterns that have a significant impact on sales success.
For example, companies can determine which products are selling particularly well and which are less successful. In addition, forecasts on cross-selling, customer churn and pricing margins can be made.
In addition, forecasts on cross-selling, Customer churn and pricing margins can also be determined with this data. This data source is particularly suitable for predictive analytics.
– Customer data (CRM data)
Customer data is usually stored in a CRM system.In most cases, sales activities and customer information are documented in the CRM systems by the sales teams. This takes place both during customer visits and during telephone calls.
This means that the “data”, i.e. the information, varies greatly. It includes information on demographic characteristics, purchasing behavior, preferences and customer feedback.
The disadvantage of CRM data is the high level of effort involved in standardizing this large amount of contextual data. For most data analyses, it is necessary for the data to be available in a standardized form.
However, collecting and analyzing this data makes it possible to better understand your own target groups and adapt marketing and sales strategies accordingly.
Personalized offers and communication tailored to the respective customers lead to higher customer satisfaction and customer loyalty.
– External (market) data
External data includes information on market conditions, social media, competition and industry trends.
This data is very tempting and sought-after. However, obtaining this data is anything but easy and involves major challenges. In addition, the reliability of this data is often questionable.
With your own sales data, you can be almost 100% sure that this data is correct and that new, valid data is regularly added. On the other hand, if you have a competitor’s price list that is two years old, you can only derive limited benefit from it in terms of data analysis. Market data, such as the gross national product of various countries, is also never as up-to-date as your own sales data.
Knowledge of external market data is undeniably important for strategic business decisions at the highest level.
However, we advise against using this data source for precise sales forecasts. The data is not reliable and the cost-benefit ratio is not profitable.
Methods of data analysis in sales
Data analysis in sales can be carried out in various ways, depending on the specific goals and requirements of the company:
– Descriptive analysis
Descriptive analysis focuses on describing and visualizing existing data. It answers questions such as “What happened?” and uses tools such as dashboards and reports to illustrate data patterns and trends. This method is ideal for gaining an initial overview of the sales situation and recognizing basic patterns.
– Diagnostic analysis
Diagnostic analysis goes one step further and aims to identify the causes behind the observed patterns and trends.
It provides answers to questions such as “Why did this happen?” and uses statistical methods to identify relationships and correlations.
The results of this analysis provide valuable insights into the factors that influence sales success.
– Predictive analysis (predictive analytics)
Predictive analytics uses historical data and machine learning algorithms to predict future sales trends and results.It answers questions such as “What is likely to happen?”.This method is particularly valuable for developing proactive sales strategies and preparing for future challenges and opportunities.
– Prescriptive analysis
Prescriptive analysis is a more advanced method that not only makes predictions but also provides specific recommendations for action. It answers questions such as “What should we do?” and uses advanced algorithms to make optimal decisions. This method enables companies to optimize their sales strategies and make automated decisions based on data.
For example, Qymatix Predictive Sales Software provides sales teams with concrete recommendations for action based on predictive analyses. It is therefore a good example of the use of prescriptive analysis.
Best practices for the optimal use of data analytics in sales
To realize the full potential of data analytics, companies should follow some best practices:
– Start small
Start with what you have – your own sales data! You can use Excel to gain initial insights from your data, such as cross-selling potential or customers at risk of churning.
If you find that implementation is too time-consuming, Qymatix is a provider of predictive sales software. This gives you AI-based recommendations for action directly for your sales team.
– Data quality and maintenance
High data quality is crucial for effective data analysis. Companies are obliged to ensure that their data is accurate, complete and up-to-date. Regular data maintenance and updating is essential to achieve reliable analysis results. Incorrect or outdated data can lead to incorrect conclusions and suboptimal decisions.
If you work with a reliable provider, experts will look at your data quality and can assess whether it is suitable for the use of AI or whether there is still potential for optimization.
– Training and further education
Only when sales teams understand the importance and use of data analysis results can they effectively integrate the insights gained into their daily work processes.
They should be given concrete approaches and ideas on how to apply data-based results to customers without getting into awkward situations.
CALCULATE NOW THE ROI OF QYMATIX PREDICTIVE SALES SOFTWARE
Collaboration between departments
Close collaboration between sales, marketing and IT is essential in order to take full advantage of data analysis. These departments should work together to collect, analyze and interpret data in order to obtain a holistic picture of the sales situation. An integrated approach promotes the sharing of data and insights and supports the development of coherent and effective strategies.